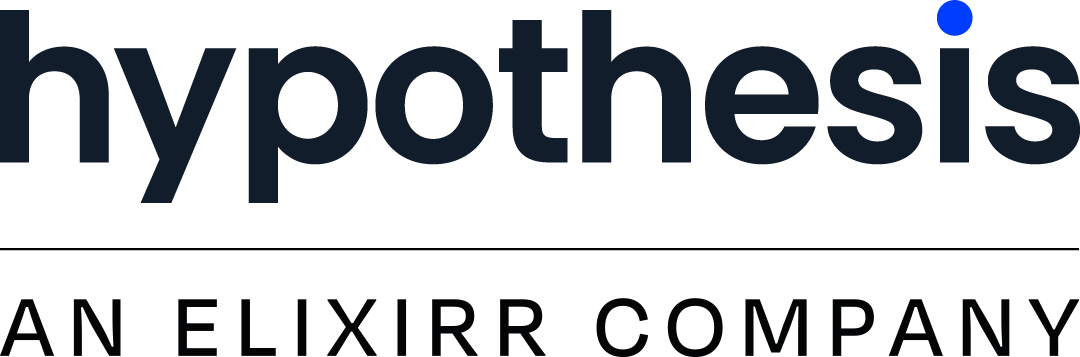

Insights . Strategy . Design .
We help important brands do amazing things., hypothesis combines insights, strategy, and design to help brands unlock new opportunities..
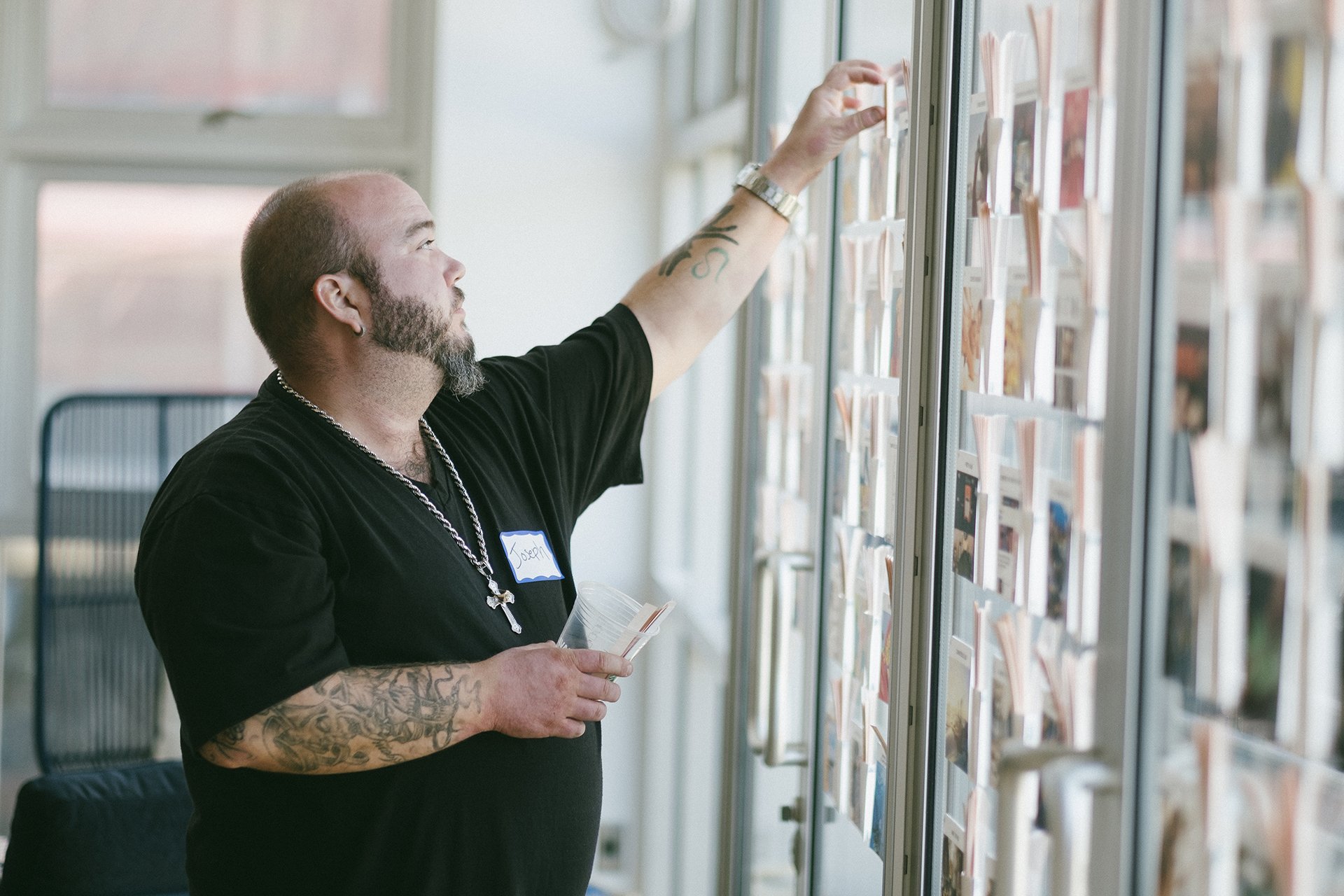
Understand audiences and attract new customers
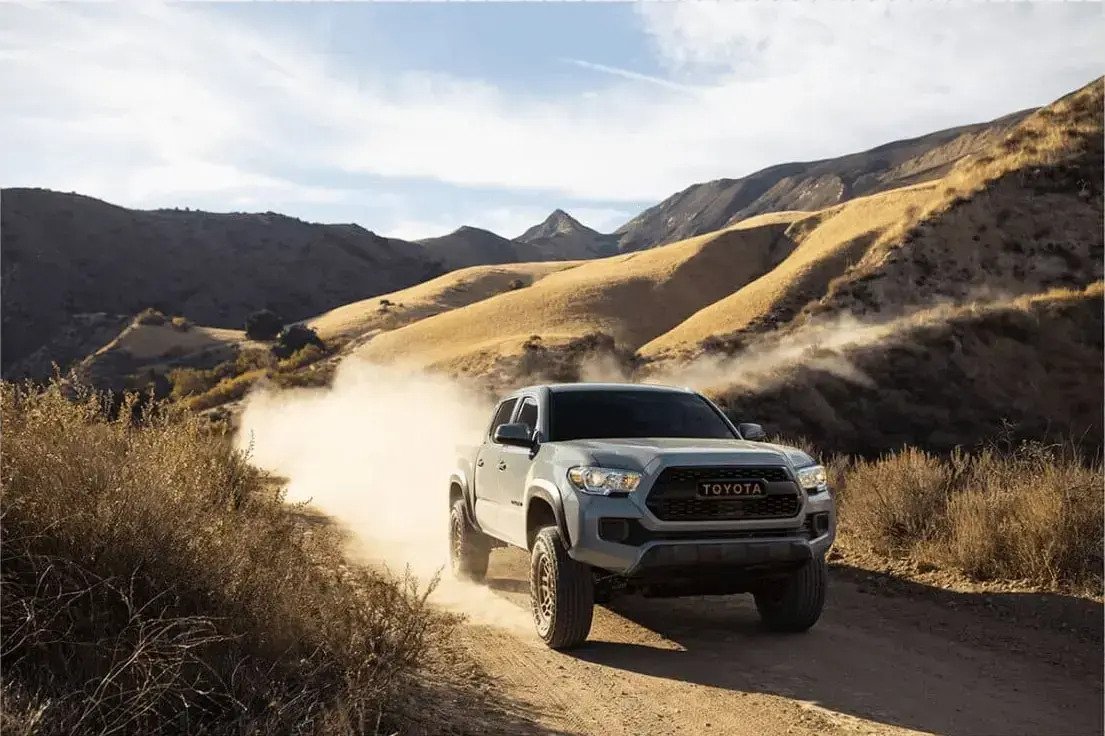
Position, build, and strengthen brands to thrive
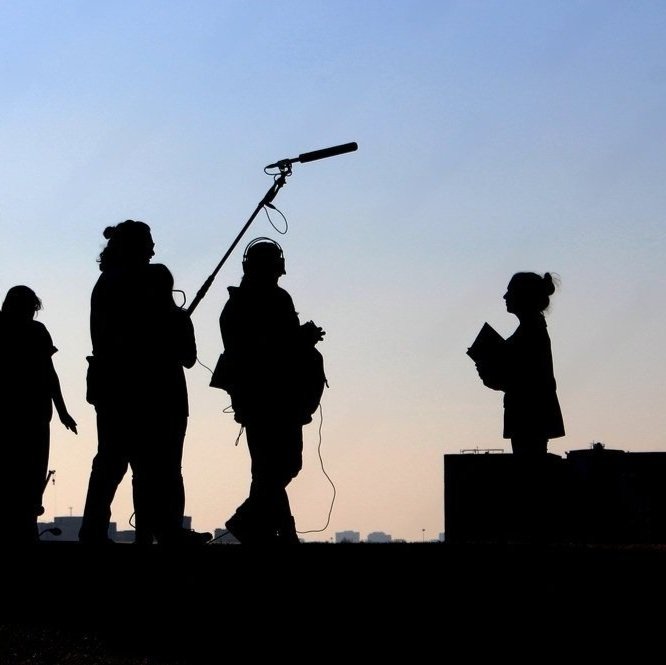
Create content and communications audiences love
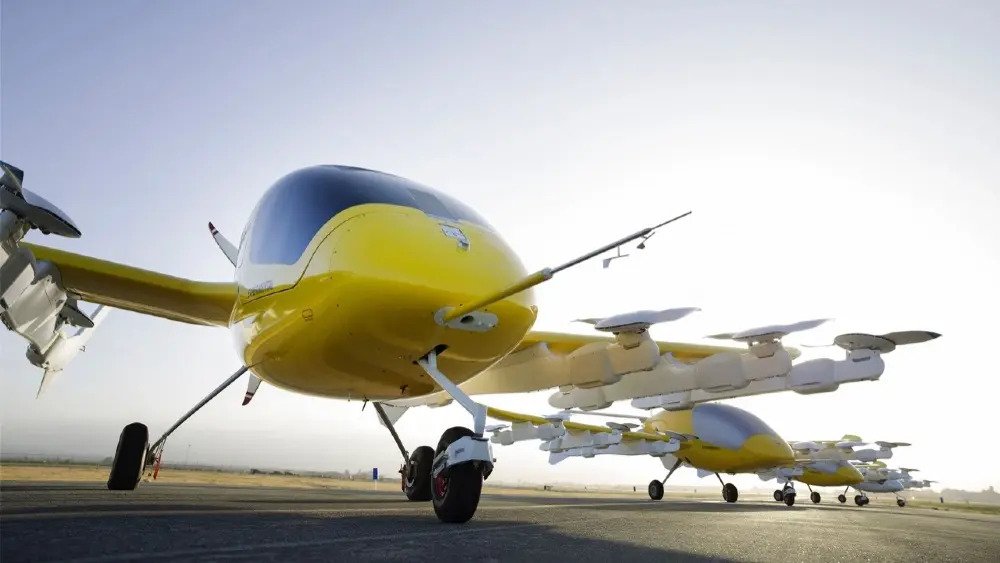
Develop products and services that deliver growth
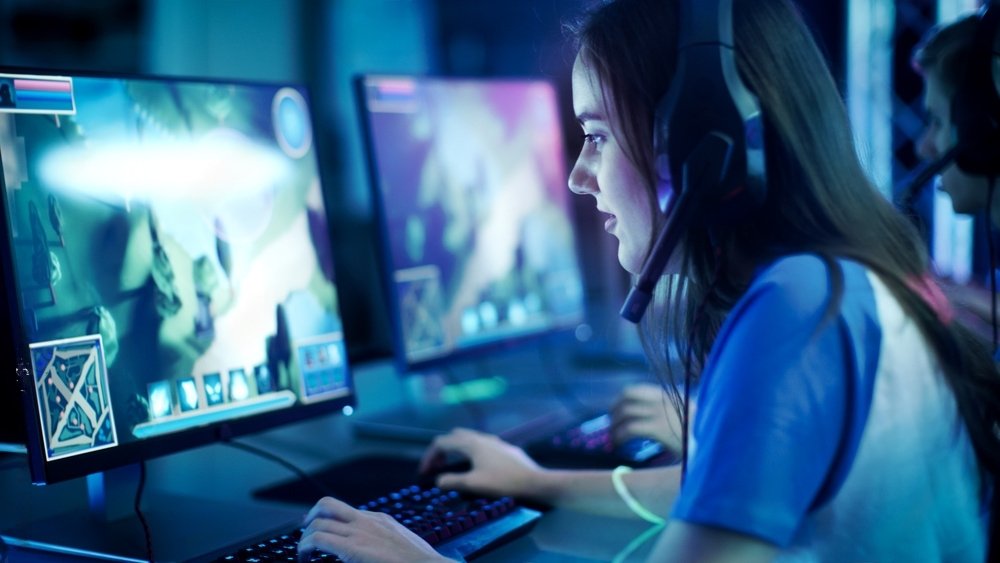
Purpose & Culture
Operate with purpose and create values-driven culture
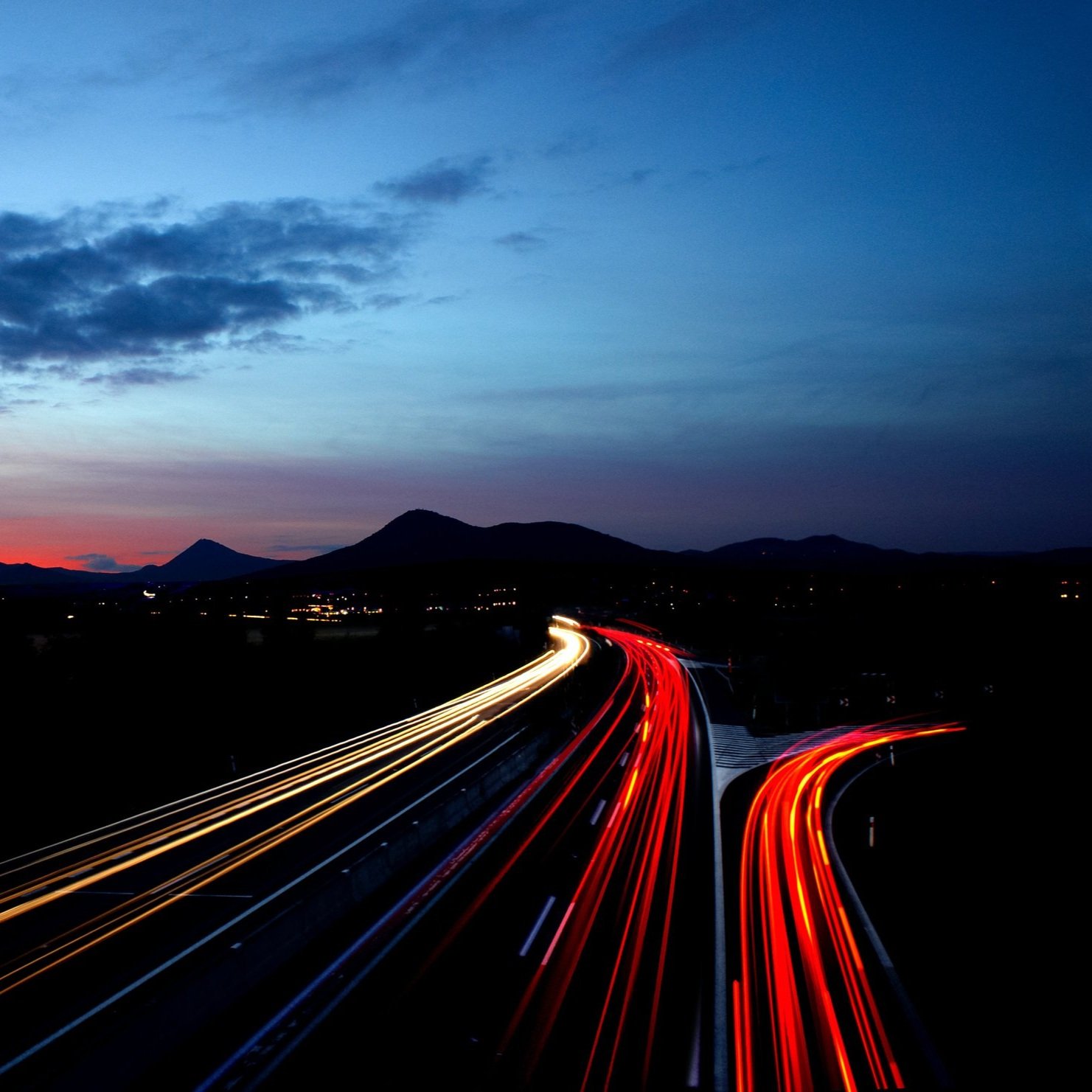
Future States
Capitalize on emerging trends to find opportunity
CAPABILITIES
That “just right” mix of experts and expertise. .
We pair highly experienced people with creative approaches to help brands figure out where go next, and how to get there.
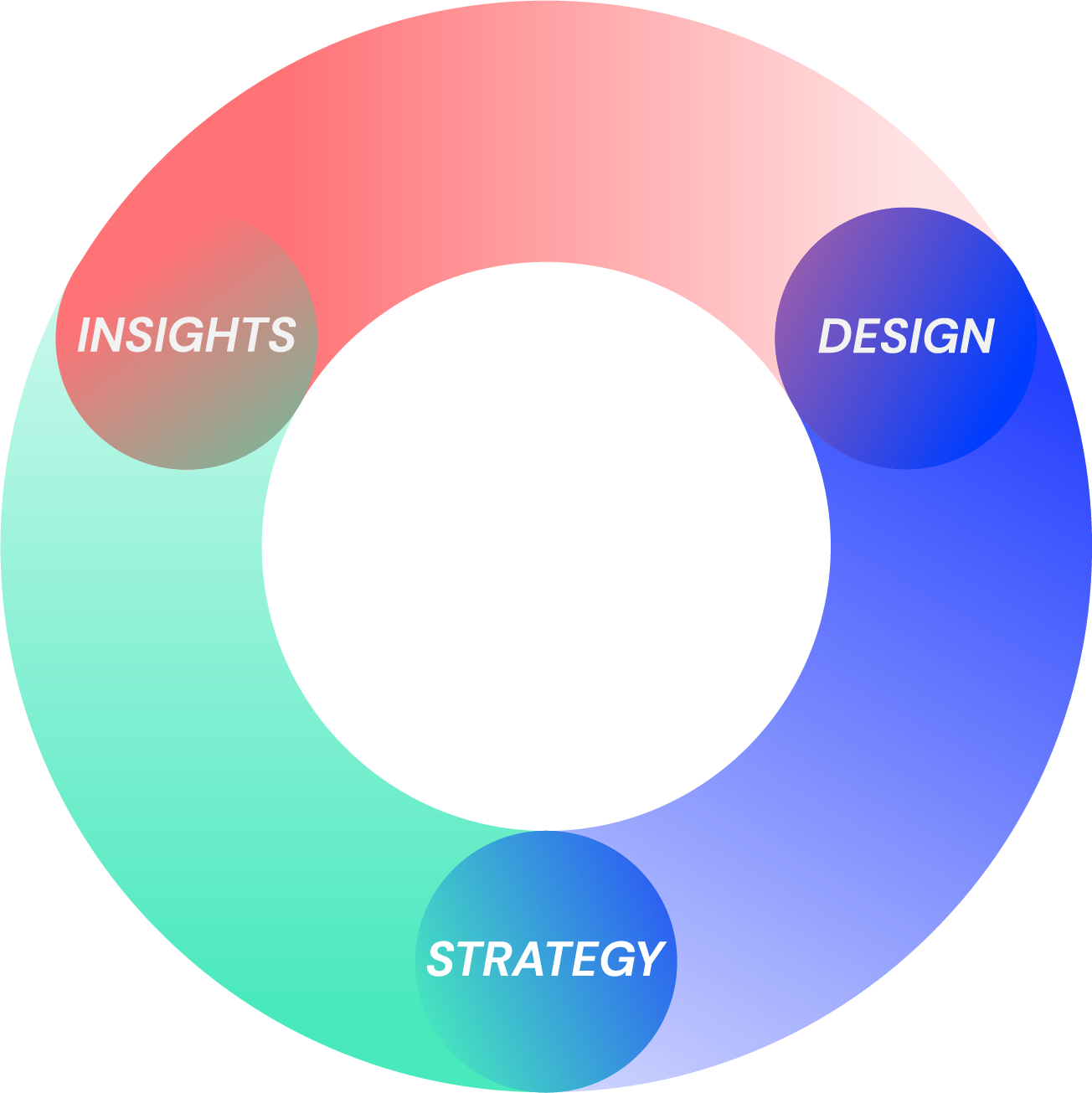
We’re with you for the long haul.
When your insights partner "gets" your brand inside and out, amazing things happen. We build lasting relationships with our clients, taking a big picture view of your business goals with every project.
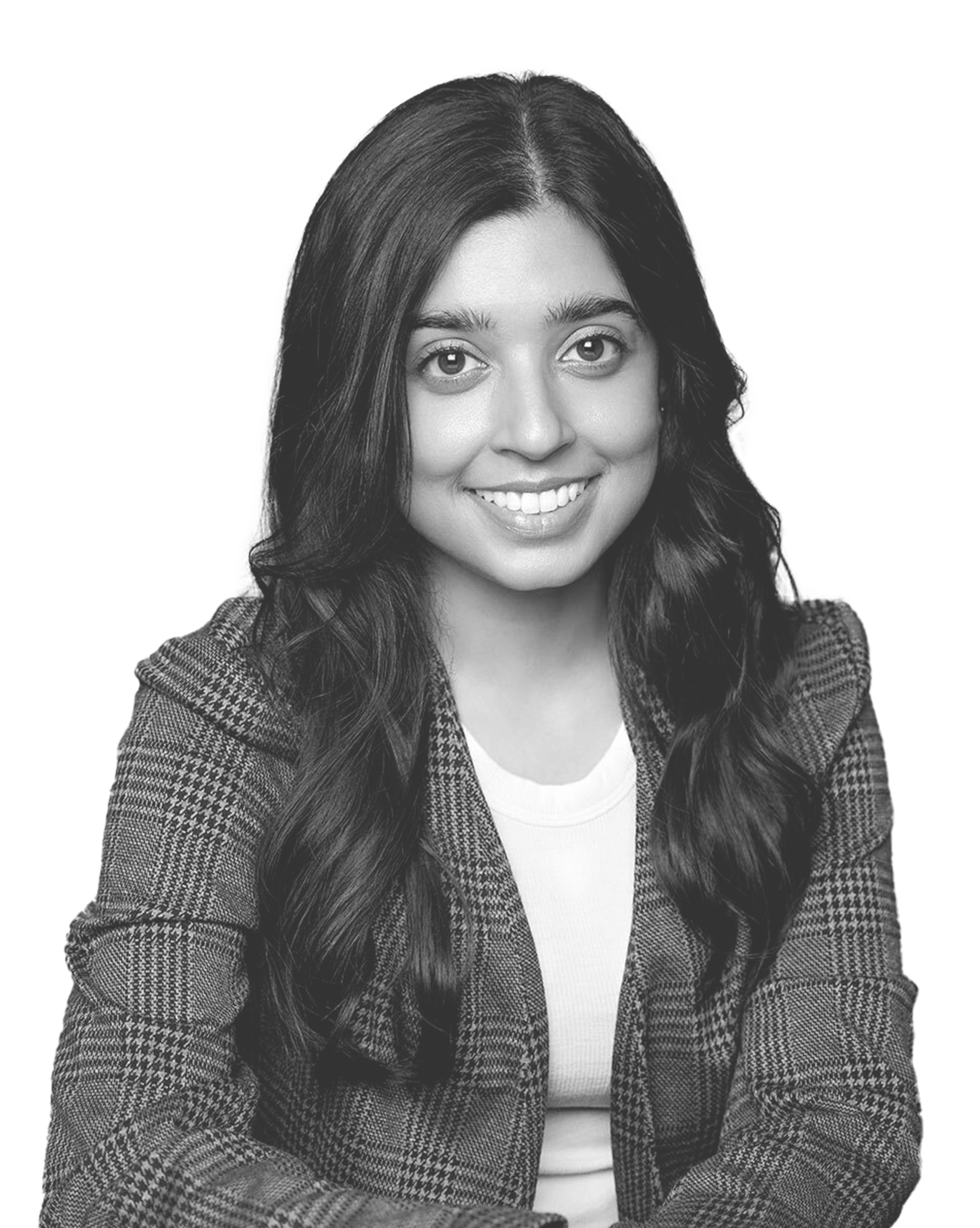
Let’s work together.
Our work in action.
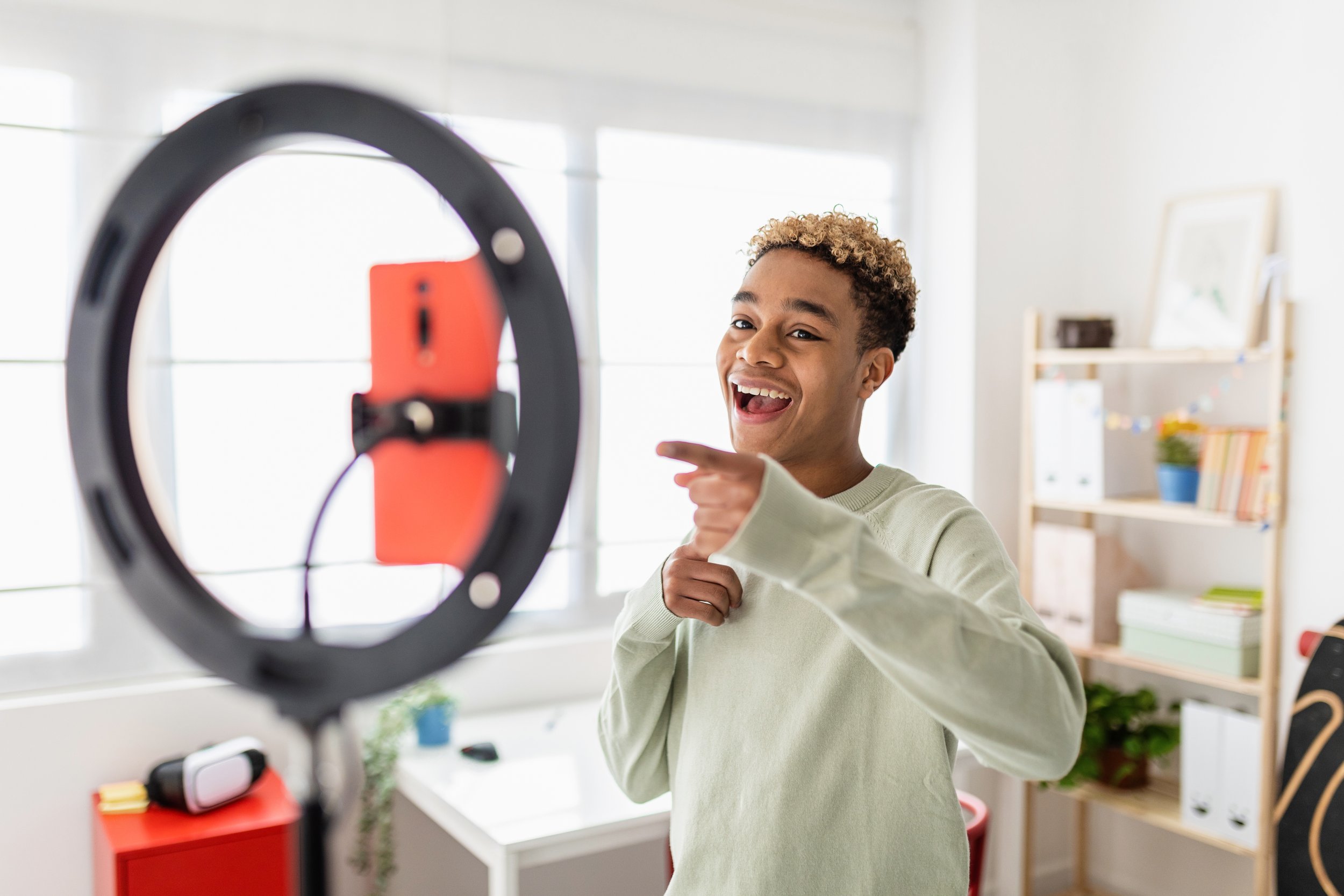
Creating space for teen trust and safety online.
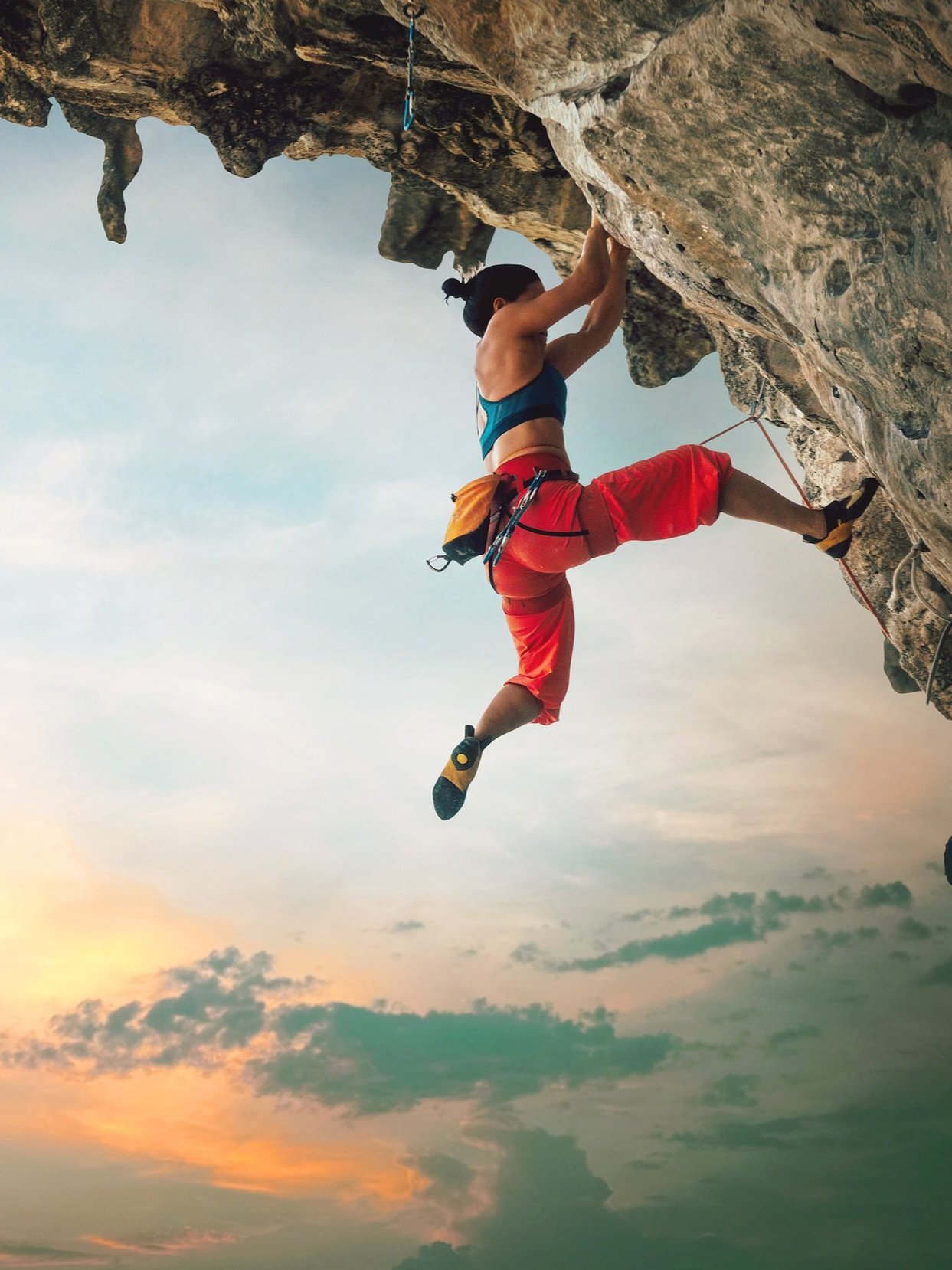
NATIONAL GEOGRAPHIC
Refreshing a heritage brand for the next generation.
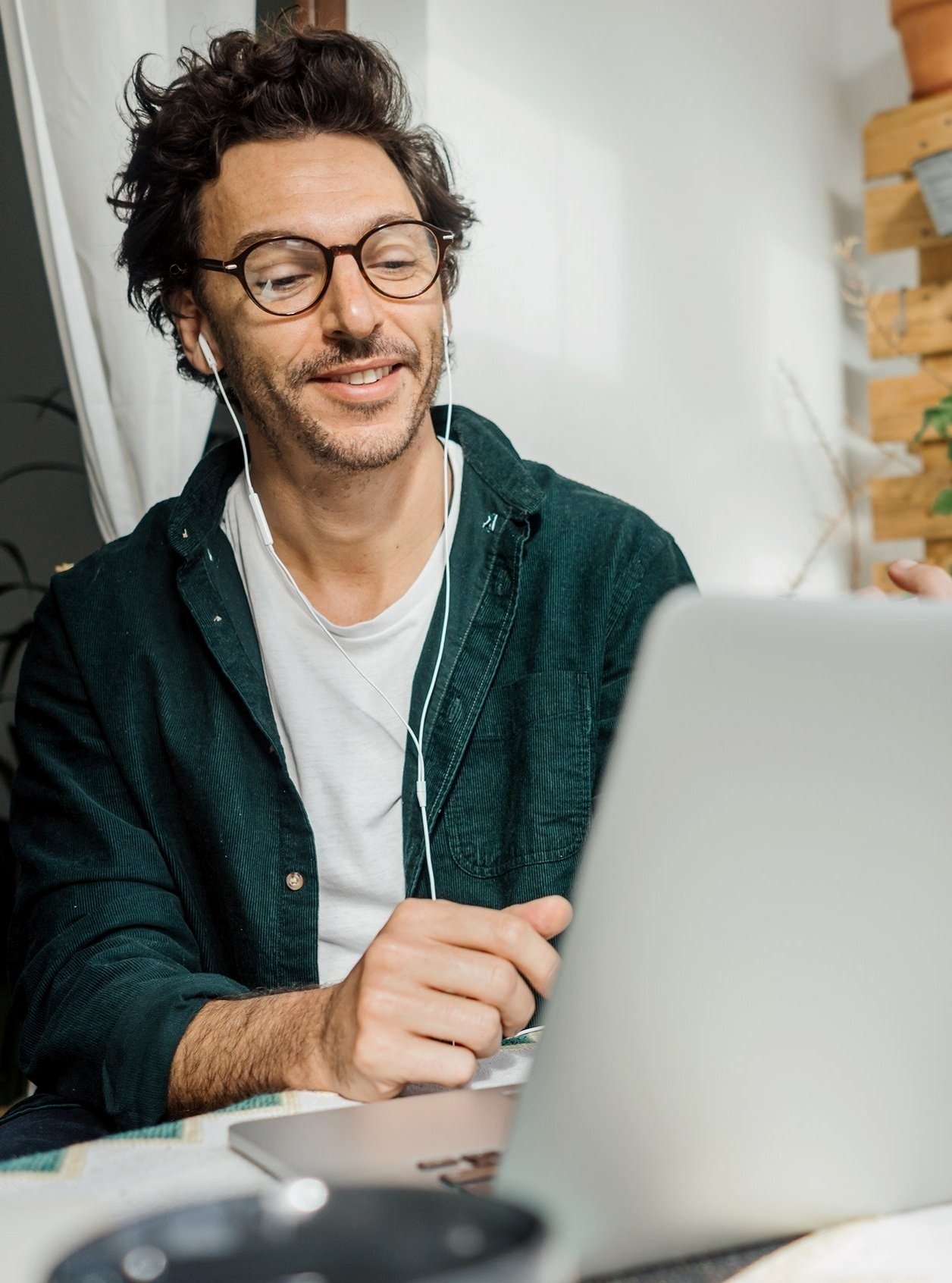
Planning new technology for the future of work.
Our Clients
Judge us by the companies we keep. .
Our clients are among the most innovative, dynamic, and admired brands in the world. We’re thrilled by their success and proud that they continue to turn to us for their next big move.
Meet the Team
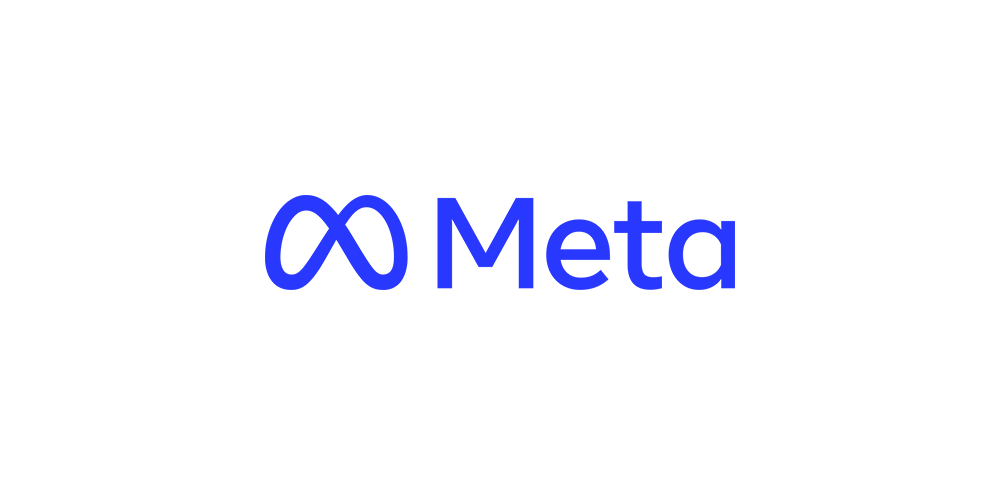
Client LOVE
This is the crown jewel of the research team's work for the year. the entire company is building their strategy around this., i've worked with so many agencies in the past, and you all are at the very top., we are getting great reception from our stakeholders... this work will have a big impact., this research is now the gold standard of qual at (our company)., it was so easy for me to communicate with you guys. i always knew who was in charge of each part and felt the whole team was one., it is a pleasure to work with your whole team on every single project., this is fantastic - magnificently designed, really cool insights, will definitely get people's attention., you guys have data viz down more than any other vendor i work with... it's clean, low data density, and persuasive., we’ve been so impressed with the rigor and flexibility you've shown all along., featured honors.
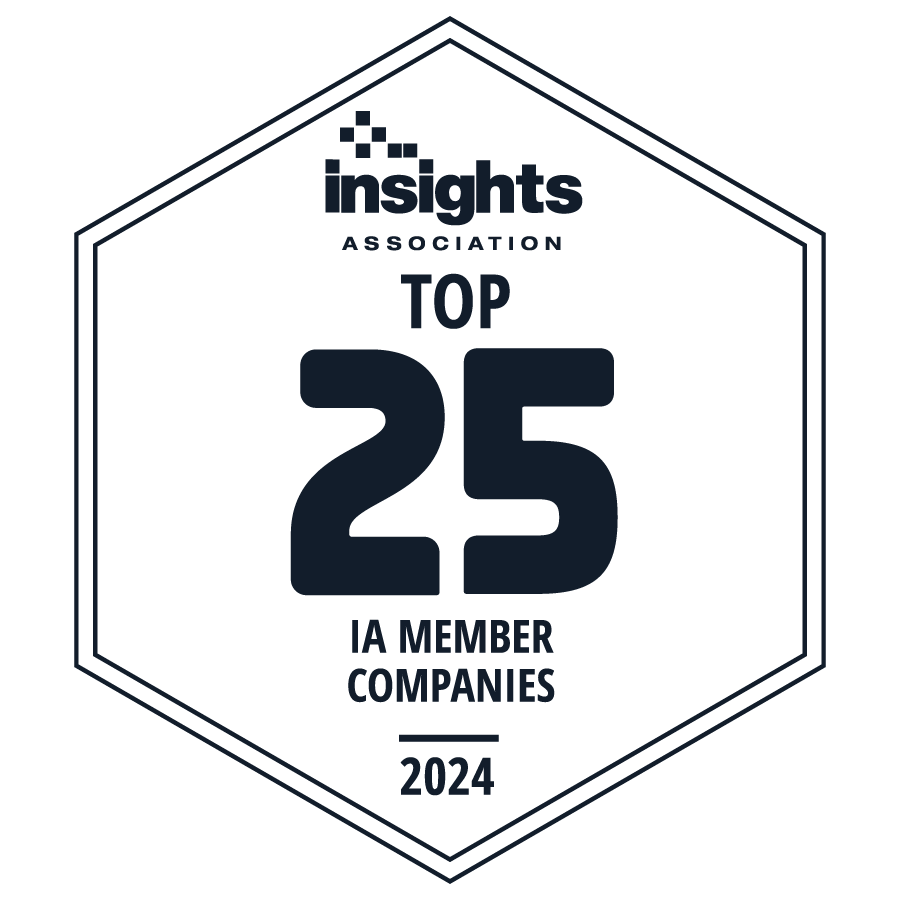
HYPOTHESIS TAKES DOUBLE GOLD AT OGILVY AWARDS
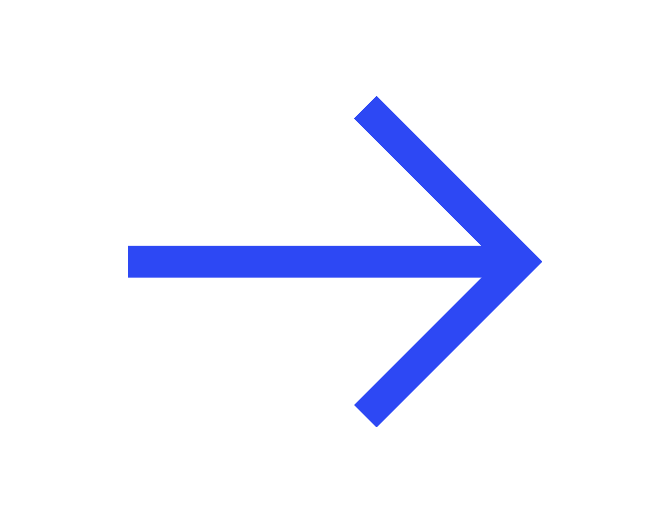
HYPOTHESIS WINS SECOND HOW DESIGN AWARD
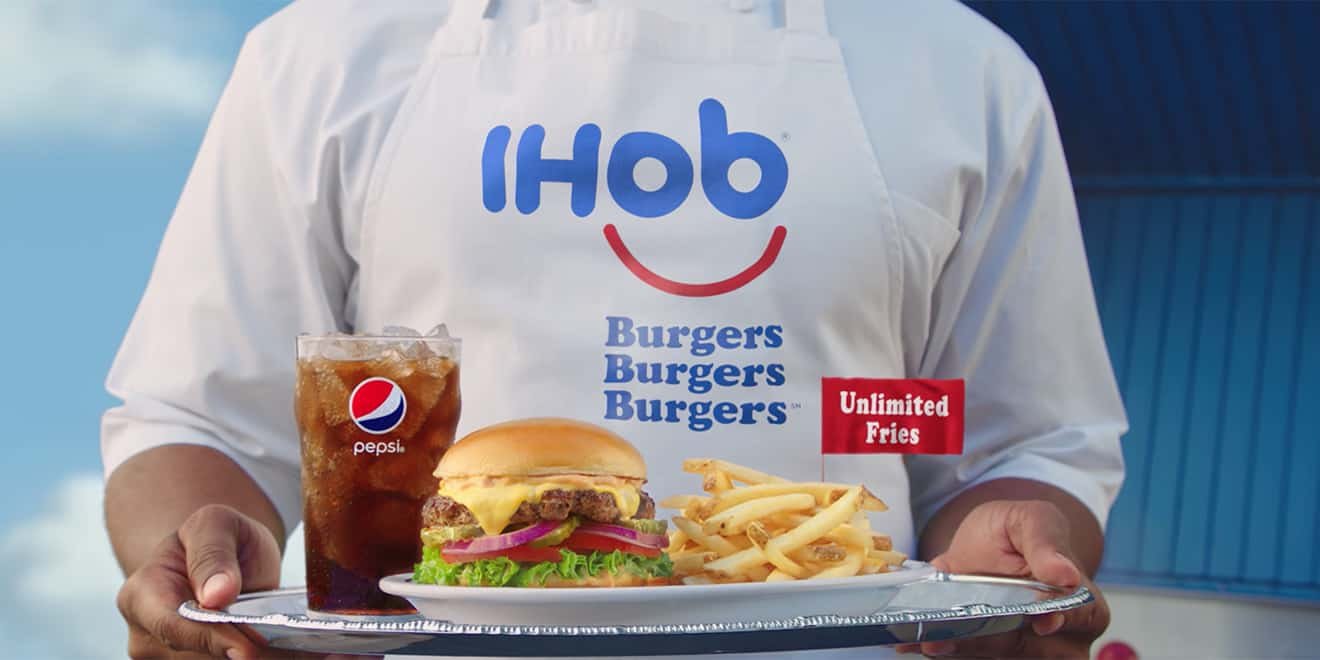
HYPOTHESIS AND IHOP SCORE TRIPLE WIN, INCLUDING GRAND OGILVY AWARD
Do career-defining work with amazing people..
We’re looking for smart, passionate individuals with unique backgrounds who like to collaborate and love a good story. Sound like you? Apply to one of our open positions and see what all the Hyp is about.
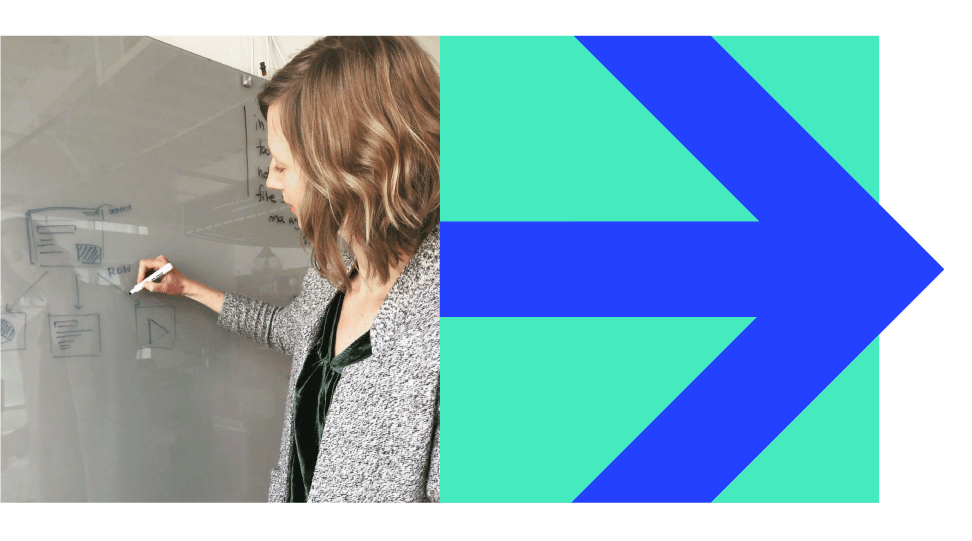
Let's Stay Connected
Sign up to receive news and updates.
Thanks for signing up! Stay tuned for more updates.
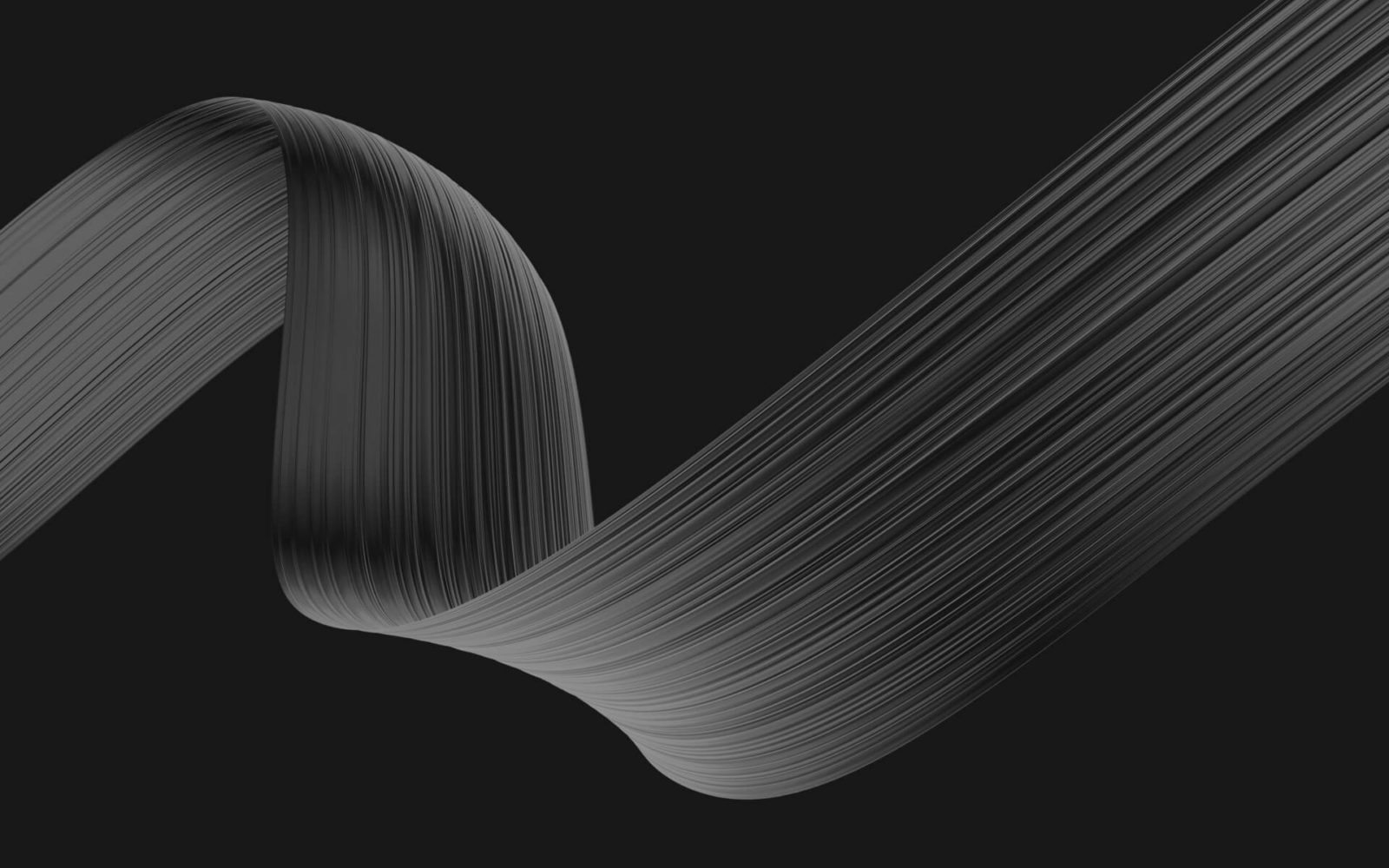
How to write a hypothesis for marketing experimentation
- Apr 11, 2021
- 5 minute read
- Creating your strongest marketing hypothesis
The potential for your marketing improvement depends on the strength of your testing hypotheses.
But where are you getting your test ideas from? Have you been scouring competitor sites, or perhaps pulling from previous designs on your site? The web is full of ideas and you’re full of ideas – there is no shortage of inspiration, that’s for sure.
Coming up with something you want to test isn’t hard to do. Coming up with something you should test can be hard to do.
Hard – yes. Impossible? No. Which is good news, because if you can’t create hypotheses for things that should be tested, your test results won’t mean mean much, and you probably shouldn’t be spending your time testing.
Taking the time to write your hypotheses correctly will help you structure your ideas, get better results, and avoid wasting traffic on poor test designs.
With this post, we’re getting advanced with marketing hypotheses, showing you how to write and structure your hypotheses to gain both business results and marketing insights!
By the time you finish reading, you’ll be able to:
- Distinguish a solid hypothesis from a time-waster, and
- Structure your solid hypothesis to get results and insights
To make this whole experience a bit more tangible, let’s track a sample idea from…well…idea to hypothesis.
Let’s say you identified a call-to-action (CTA)* while browsing the web, and you were inspired to test something similar on your own lead generation landing page. You think it might work for your users! Your idea is:
“My page needs a new CTA.”
*A call-to-action is the point where you, as a marketer, ask your prospect to do something on your page. It often includes a button or link to an action like “Buy”, “Sign up”, or “Request a quote”.
The basics: The correct marketing hypothesis format
Level up: moving from a good to great hypothesis, it’s based on a science, building marketing hypotheses to create insights, what makes a great hypothesis.
A well-structured hypothesis provides insights whether it is proved, disproved, or results are inconclusive.
You should never phrase a marketing hypothesis as a question. It should be written as a statement that can be rejected or confirmed.
Further, it should be a statement geared toward revealing insights – with this in mind, it helps to imagine each statement followed by a reason :
- Changing _______ into ______ will increase [conversion goal], because:
- Changing _______ into ______ will decrease [conversion goal], because:
- Changing _______ into ______ will not affect [conversion goal], because:
Each of the above sentences ends with ‘because’ to set the expectation that there will be an explanation behind the results of whatever you’re testing.
It’s important to remember to plan ahead when you create a test, and think about explaining why the test turned out the way it did when the results come in.
Understanding what makes an idea worth testing is necessary for your optimization team.
If your tests are based on random ideas you googled or were suggested by a consultant, your testing process still has its training wheels on. Great hypotheses aren’t random. They’re based on rationale and aim for learning.
Hypotheses should be based on themes and analysis that show potential conversion barriers.
At Conversion, we call this investigation phase the “Explore Phase” where we use frameworks like the LIFT Model to understand the prospect’s unique perspective. (You can read more on the the full optimization process here).
A well-founded marketing hypothesis should also provide you with new, testable clues about your users regardless of whether or not the test wins, loses or yields inconclusive results.
These new insights should inform future testing: a solid hypothesis can help you quickly separate worthwhile ideas from the rest when planning follow-up tests.
“Ultimately, what matters most is that you have a hypothesis going into each experiment and you design each experiment to address that hypothesis.” – Nick So, VP of Delivery
Here’s a quick tip :
If you’re about to run a test that isn’t going to tell you anything new about your users and their motivations, it’s probably not worth investing your time in.
Let’s take this opportunity to refer back to your original idea:
Ok, but what now ? To get actionable insights from ‘a new CTA’, you need to know why it behaved the way it did. You need to ask the right question.
To test the waters, maybe you changed the copy of the CTA button on your lead generation form from “Submit” to “Send demo request”. If this change leads to an increase in conversions, it could mean that your users require more clarity about what their information is being used for.
That’s a potential insight.
Based on this insight, you could follow up with another test that adds copy around the CTA about next steps: what the user should anticipate after they have submitted their information.
For example, will they be speaking to a specialist via email? Will something be waiting for them the next time they visit your site? You can test providing more information, and see if your users are interested in knowing it!
That’s the cool thing about a good hypothesis: the results of the test, while important (of course) aren’t the only component driving your future test ideas. The insights gleaned lead to further hypotheses and insights in a virtuous cycle.
The term “hypothesis” probably isn’t foreign to you. In fact, it may bring up memories of grade-school science class; it’s a critical part of the scientific method .
The scientific method in testing follows a systematic routine that sets ideation up to predict the results of experiments via:
- Collecting data and information through observation
- Creating tentative descriptions of what is being observed
- Forming hypotheses that predict different outcomes based on these observations
- Testing your hypotheses
- Analyzing the data, drawing conclusions and insights from the results
Don’t worry! Hypothesizing may seem ‘sciency’, but it doesn’t have to be complicated in practice.
Hypothesizing simply helps ensure the results from your tests are quantifiable, and is necessary if you want to understand how the results reflect the change made in your test.
A strong marketing hypothesis allows testers to use a structured approach in order to discover what works, why it works, how it works, where it works, and who it works on.
“My page needs a new CTA.” Is this idea in its current state clear enough to help you understand what works? Maybe. Why it works? No. Where it works? Maybe. Who it works on? No.
Your idea needs refining.
Let’s pull back and take a broader look at the lead generation landing page we want to test.
Imagine the situation: you’ve been diligent in your data collection and you notice several recurrences of Clarity pain points – meaning that there are many unclear instances throughout the page’s messaging.
Rather than focusing on the CTA right off the bat, it may be more beneficial to deal with the bigger clarity issue.
Now you’re starting to think about solving your prospects conversion barriers rather than just testing random ideas!
If you believe the overall page is unclear, your overarching theme of inquiry might be positioned as:
- “Improving the clarity of the page will reduce confusion and improve [conversion goal].”
By testing a hypothesis that supports this clarity theme, you can gain confidence in the validity of it as an actionable marketing insight over time.
If the test results are negative : It may not be worth investigating this motivational barrier any further on this page. In this case, you could return to the data and look at the other motivational barriers that might be affecting user behavior.
If the test results are positive : You might want to continue to refine the clarity of the page’s message with further testing.
Typically, a test will start with a broad idea — you identify the changes to make, predict how those changes will impact your conversion goal, and write it out as a broad theme as shown above. Then, repeated tests aimed at that theme will confirm or undermine the strength of the underlying insight.
You believe you’ve identified an overall problem on your landing page (there’s a problem with clarity). Now you want to understand how individual elements contribute to the problem, and the effect these individual elements have on your users.
It’s game time – now you can start designing a hypothesis that will generate insights.
You believe your users need more clarity. You’re ready to dig deeper to find out if that’s true!
If a specific question needs answering, you should structure your test to make a single change. This isolation might ask: “What element are users most sensitive to when it comes to the lack of clarity?” and “What changes do I believe will support increasing clarity?”
At this point, you’ll want to boil down your overarching theme…
- Improving the clarity of the page will reduce confusion and improve [conversion goal].
…into a quantifiable hypothesis that isolates key sections:
- Changing the wording of this CTA to set expectations for users (from “submit” to “send demo request”) will reduce confusion about the next steps in the funnel and improve order completions.
Does this answer what works? Yes: changing the wording on your CTA.
Does this answer why it works? Yes: reducing confusion about the next steps in the funnel.
Does this answer where it works? Yes: on this page, before the user enters this theoretical funnel.
Does this answer who it works on? No, this question demands another isolation. You might structure your hypothesis more like this:
- Changing the wording of the CTA to set expectations for users (from “submit” to “send demo request”) will reduce confusion for visitors coming from my email campaign about the next steps in the funnel and improve order completions.
Now we’ve got a clear hypothesis. And one worth testing!
1. It’s testable.
2. It addresses conversion barriers.
3. It aims at gaining marketing insights.
Let’s compare:
The original idea : “My page needs a new CTA.”
Following the hypothesis structure : “A new CTA on my page will increase [conversion goal]”
The first test implied a problem with clarity, provides a potential theme : “Improving the clarity of the page will reduce confusion and improve [conversion goal].”
The potential clarity theme leads to a new hypothesis : “Changing the wording of the CTA to set expectations for users (from “submit” to “send demo request”) will reduce confusion about the next steps in the funnel and improve order completions.”
Final refined hypothesis : “Changing the wording of the CTA to set expectations for users (from “submit” to “send demo request”) will reduce confusion for visitors coming from my email campaign about the next steps in the funnel and improve order completions.”
Which test would you rather your team invest in?
Before you start your next test, take the time to do a proper analysis of the page you want to focus on. Do preliminary testing to define bigger issues, and use that information to refine and pinpoint your marketing hypothesis to give you forward-looking insights.
Doing this will help you avoid time-wasting tests, and enable you to start getting some insights for your team to keep testing!
Share this post
Other articles you might like
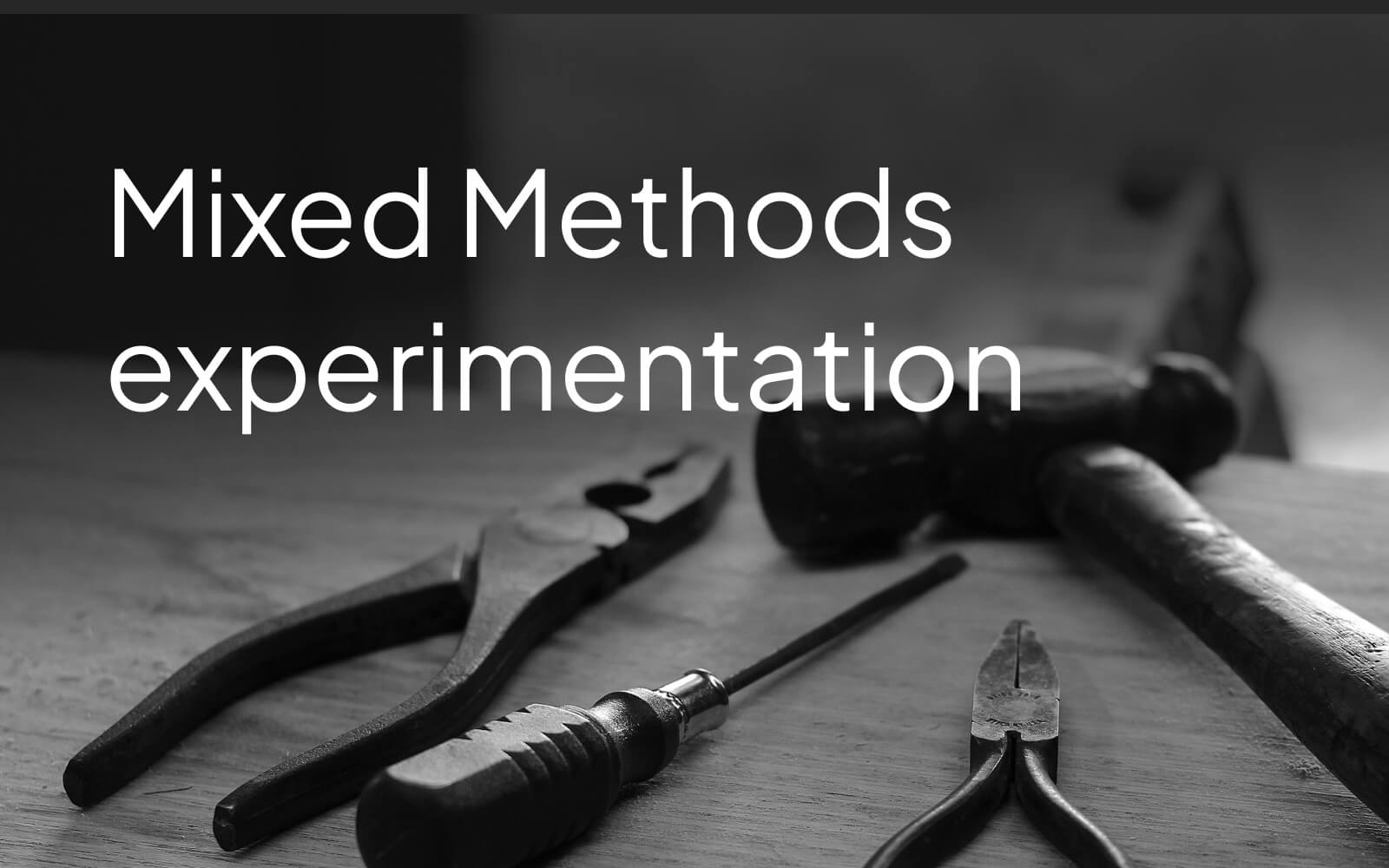
Mixed Methods Experimentation
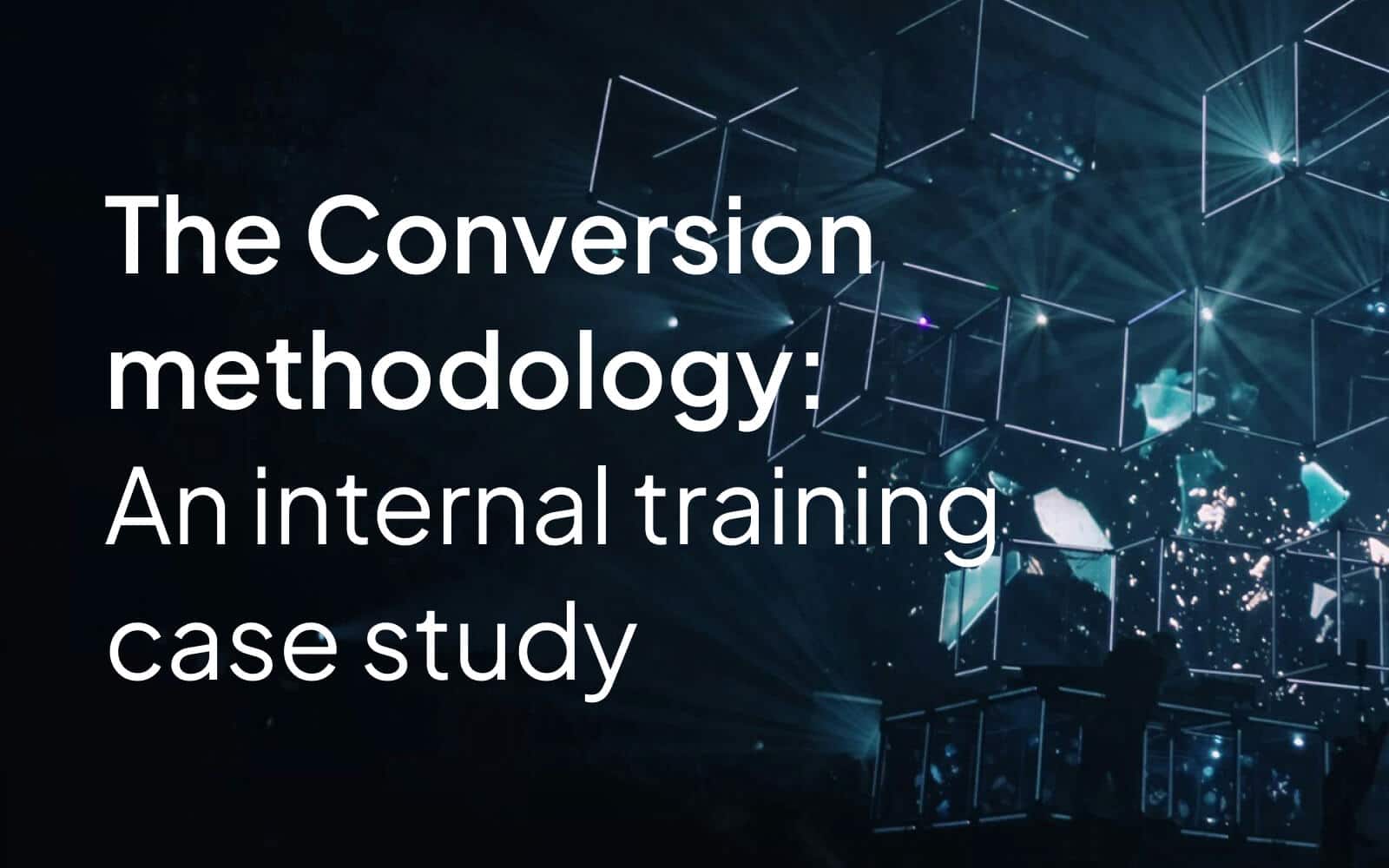
The Conversion Methodology: an internal training case study
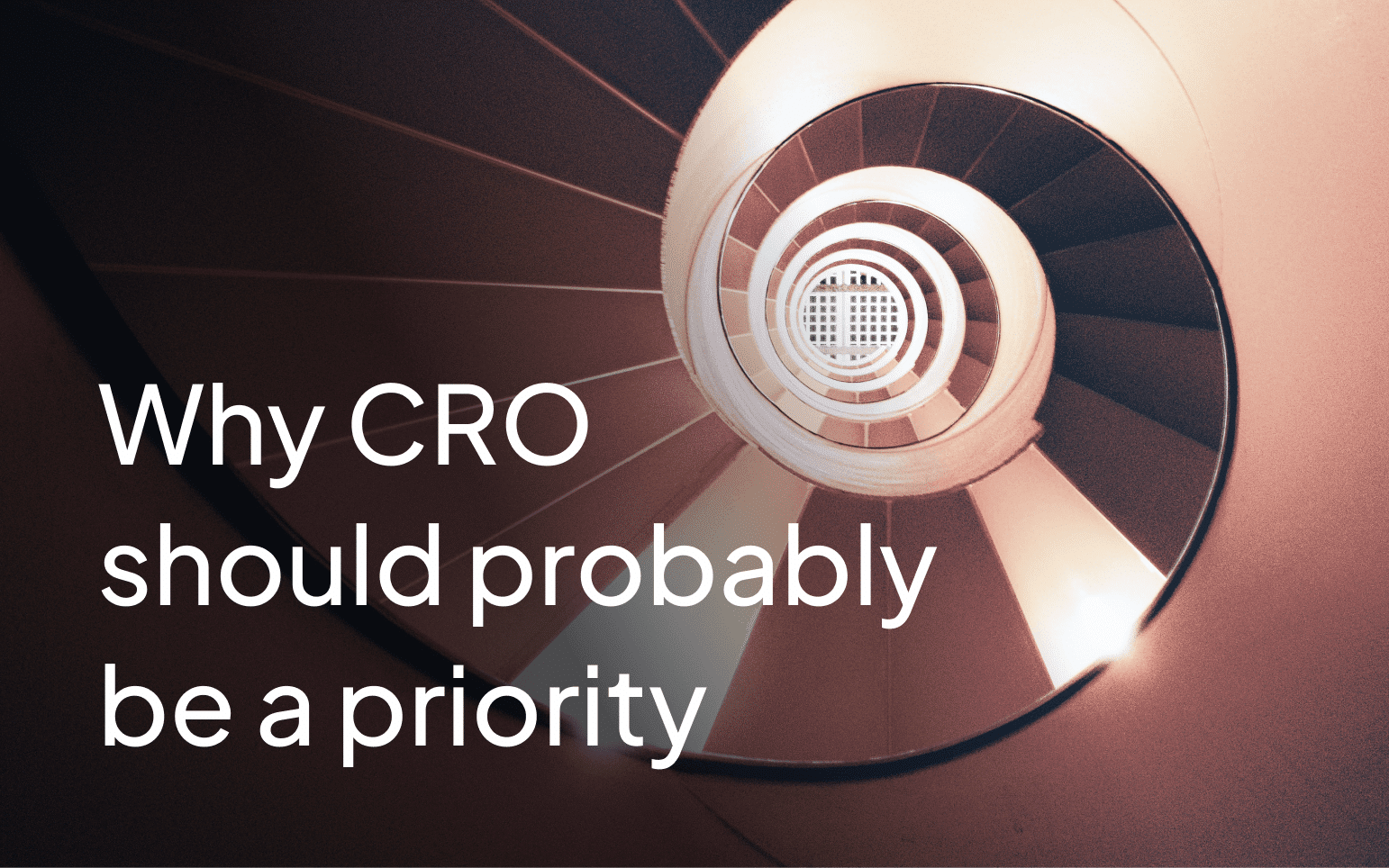
Why CRO should (probably) be a priority
Hypothesis testing: Hypothesis Testing in Market Research Methodology: A Beginner's Guide
1. introduction to hypothesis testing in market research methodology, 2. understanding the basics of hypothesis testing, 3. formulating a hypothesis in market research, 4. types of hypothesis testing in market research, 5. step-by-step guide, 6. interpreting the results of a hypothesis test, 7. common pitfalls and challenges in hypothesis testing, 8. best practices for effective hypothesis testing in market research, 9. harnessing the power of hypothesis testing in market research.
In the realm of market research, hypothesis testing is a crucial tool that enables researchers to make informed decisions based on data analysis . It allows us to evaluate the validity of assumptions or claims made about a population, providing valuable insights into consumer behavior , market trends, and business strategies. In this section, we will delve into the fundamentals of hypothesis testing and explore its application in market research methodology .
2. The Basics of Hypothesis Testing
Hypothesis testing involves formulating two competing hypotheses: the null hypothesis (H0) and the alternative hypothesis (Ha). The null hypothesis assumes that there is no significant difference or relationship between variables , while the alternative hypothesis posits the existence of a significant difference or relationship. Through statistical analysis, we aim to either accept or reject the null hypothesis based on the evidence provided by the data.
For example, a market researcher might be interested in determining whether a new advertising campaign has a significant impact on sales. The null hypothesis would state that the campaign has no effect, while the alternative hypothesis would suggest that the campaign does have an effect. By collecting data on sales before and after the campaign, the researcher can perform hypothesis testing to draw meaningful conclusions.
3. Tips for Conducting hypothesis Testing in Market research
To ensure accurate and reliable results, it is essential to follow best practices when conducting hypothesis testing in market research . Here are a few tips to keep in mind:
A. Clearly define the research question: Before formulating hypotheses, it is crucial to have a clear understanding of the research question. Clearly defining the objectives and variables under investigation will help guide the hypothesis formulation process.
B. Select appropriate statistical tests: Different research questions require different statistical tests. Understanding the nature of the data and the relationship between variables will aid in selecting the most suitable test for hypothesis testing . Common tests include t-tests, chi-square tests , and ANOVA.
C. Determine the significance level: The significance level, denoted as , determines the threshold for accepting or rejecting the null hypothesis. Commonly used values for are 0.05 and 0.01, indicating a 5% and 1% chance of making a Type I error, respectively.
D. Collect sufficient sample size: adequate sample size is crucial for obtaining reliable results. A small sample size may not provide enough statistical power to detect significant differences accurately. Calculating sample size requirements prior to data collection is recommended.
4. Case Study: Hypothesis Testing in Market Research
To illustrate the practical application of hypothesis testing in market research, let's consider a case study. A company wishes to assess whether a new packaging design for their product will increase customer satisfaction . They randomly select two groups of customers, one exposed to the new packaging and the other to the old packaging. After collecting feedback from both groups, they perform a hypothesis test to compare the mean satisfaction ratings.
The null hypothesis states that there is no difference in customer satisfaction between the two packaging designs, while the alternative hypothesis suggests that the new packaging leads to higher satisfaction levels . By analyzing the collected data using a suitable statistical test (e.g., a two-sample t-test), the company can determine whether the new packaging design has a significant impact on customer satisfaction .
In conclusion, hypothesis testing plays a vital role in market research methodology by providing a systematic approach to evaluate claims and assumptions. By formulating clear hypotheses, selecting appropriate statistical tests, and following best practices, researchers can derive meaningful insights from data analysis. Through case studies and practical examples, we have seen how hypothesis testing can be applied to assess the impact of various factors on market outcomes.
Introduction to Hypothesis Testing in Market Research Methodology - Hypothesis testing: Hypothesis Testing in Market Research Methodology: A Beginner's Guide
In the world of market research , hypothesis testing plays a crucial role in drawing meaningful conclusions from data. It allows researchers to assess the validity of assumptions and make informed decisions based on statistical evidence. Whether you're new to market research or simply looking to refresh your knowledge, understanding the basics of hypothesis testing is essential. In this section, we will delve into the key concepts and steps involved in hypothesis testing, providing you with a solid foundation to conduct effective market research .
1. Formulating a Hypothesis:
The first step in hypothesis testing is to clearly define your research question and formulate a hypothesis. A hypothesis is a statement that proposes a relationship or difference between variables. It can be either a null hypothesis (H0) or an alternative hypothesis (Ha). The null hypothesis assumes that there is no significant relationship or difference, while the alternative hypothesis suggests otherwise. For example, if you're conducting a study to determine if there is a difference in customer satisfaction between two product versions, your null hypothesis could be "There is no difference in customer satisfaction between product version A and product version B," while the alternative hypothesis would state the opposite.
2. Selecting a Significance Level:
The significance level, denoted as (alpha), determines the threshold for accepting or rejecting the null hypothesis. Commonly used significance levels are 0.05 and 0.01, indicating a 5% and 1% chance of rejecting the null hypothesis when it is true, respectively. choosing a significance level depends on the importance of making a Type I error (rejecting a true null hypothesis) versus a Type II error (failing to reject a false null hypothesis). It is crucial to select an appropriate significance level that aligns with the research objectives and the consequences of making errors.
3. collecting and Analyzing data :
Once you have formulated your hypothesis and determined the significance level, the next step is to collect relevant data and analyze it. This involves designing a study, collecting data through surveys, experiments, or other research methods, and applying appropriate statistical techniques. For instance, if you're comparing the average sales of two different marketing strategies , you can use a t-test to determine if there is a significant difference between them.
4. Calculating the Test Statistic and P-value:
In hypothesis testing, the test statistic is a numerical value that summarizes the data and allows us to assess the evidence against the null hypothesis. The choice of test statistic depends on the research question and the nature of the data. Once the test statistic is calculated, it is compared to a critical value or a p-value. The p-value is the probability of obtaining a test statistic as extreme or more extreme than the observed value, assuming the null hypothesis is true. If the p-value is less than the significance level, we reject the null hypothesis in favor of the alternative hypothesis.
5. Interpreting the Results:
After calculating the test statistic and obtaining the p-value, it is essential to interpret the results correctly. If the p-value is less than the significance level, we can conclude that there is enough evidence to reject the null hypothesis and support the alternative hypothesis . On the other hand, if the p-value is greater than the significance level, we fail to reject the null hypothesis due to insufficient evidence. It is crucial to consider the practical implications and context of the research question when interpreting the results.
- Ensure your hypothesis is specific and testable to facilitate hypothesis testing.
- Consider the sample size and statistical power to increase the reliability of your results.
- Familiarize yourself with statistical software packages to streamline the data analysis process.
Case Study:
To illustrate the application of hypothesis testing in market research, let's consider a case study. A company wants to determine if a new advertising campaign has increased brand awareness among its target audience. The null hypothesis would state that there is no difference in brand awareness before and after the campaign , while the alternative hypothesis suggests that there is an increase. By collecting data through surveys or other measurements and conducting appropriate statistical tests, the company can assess the impact of the advertising campaign on brand awareness and make data-driven decisions.
Understanding the basics of hypothesis testing equips market researchers with a powerful tool to validate assumptions, draw meaningful conclusions, and make informed business decisions . By following the steps outlined above and considering the tips and case studies , you can enhance the rigor and reliability of your market research methodology.
Understanding the Basics of Hypothesis Testing - Hypothesis testing: Hypothesis Testing in Market Research Methodology: A Beginner's Guide
In market research , formulating a hypothesis is a critical step that sets the foundation for the entire research process. A hypothesis is an educated guess or assumption about a specific aspect of the market that you want to investigate. It helps guide your research and provides a framework for collecting and analyzing data. Here are some key points to consider when formulating a hypothesis:
1. Clearly define your research objective: Before formulating a hypothesis, it is essential to clearly define what you aim to achieve through your market research . For example, if you want to understand the impact of a new advertising campaign on consumer purchasing behavior, your research objective could be to determine whether the campaign has led to an increase in sales.
2. Identify the variables: Next, identify the variables that are relevant to your research objective. Variables are the factors that you believe may influence the outcome of your research. In the advertising campaign example, variables could include factors like the duration of the campaign, the target audience, the media channels used, and the product being promoted.
3. State the relationship between variables: Once you have identified the variables, you need to state the relationship between them in your hypothesis. This relationship can be either causal or correlational. A causal hypothesis suggests that one variable directly causes changes in another variable, while a correlational hypothesis suggests that the variables are related but do not necessarily cause each other. For example, a causal hypothesis could state that increasing the duration of the advertising campaign will result in a proportional increase in sales.
4. Make your hypothesis testable: A good hypothesis should be testable, meaning that it can be proven true or false through data analysis. To ensure testability, your hypothesis should be specific, measurable, and focused on a single aspect of the market. For instance, a testable hypothesis could be: "Increasing the duration of the advertising campaign by 20% will lead to a 10% increase in sales within the target audience ."
Tips for Formulating a Hypothesis:
- Review existing literature: Before formulating your hypothesis, conduct a thorough literature review to gather insights from previous studies or research in your field. This will help you build upon existing knowledge and ensure that your hypothesis is grounded in evidence.
- Keep it simple: A hypothesis should be concise and straightforward. Avoid using complex language or including multiple variables in a single hypothesis, as this can lead to confusion and make it difficult to test.
- Be open to revisions: Formulating a hypothesis is not a one-time task. As you progress with your research, you may need to revise or refine your hypothesis based on new insights or data. Stay open-minded and flexible throughout the research process.
Case Study: Hypothesis Formulation in Market Research
Let's consider a case study to illustrate the process of formulating a hypothesis in market research . Suppose a company wants to launch a new line of skincare products targeting millennials. Their research objective is to determine whether packaging design influences millennials' purchasing decisions.
1. Research objective: To understand the impact of packaging design on millennials' purchasing decisions.
2. Variables: Packaging design, purchasing decisions.
3. Relationship: Correlational hypothesis - packaging design is related to millennials' purchasing decisions.
4. Testable hypothesis: "Skincare products with visually appealing and eco-friendly packaging designs will have a higher purchase intent among millennials compared to products with conventional packaging."
By formulating a hypothesis like this, the company can design their market research study to collect data on packaging design and millennials' purchase intent. The hypothesis will guide their analysis and help them draw meaningful conclusions about the relationship between packaging design and purchasing decisions among millennials.
In conclusion, formulating a hypothesis is a crucial step in market research as it provides a clear direction for your study. By defining your research objective, identifying variables, stating the relationship between them, and ensuring testability, you can create a strong hypothesis that guides your research efforts effectively. Remember to review existing literature, keep it simple, and be open to revisions as you progress with your research.
Formulating a Hypothesis in Market Research - Hypothesis testing: Hypothesis Testing in Market Research Methodology: A Beginner's Guide
In market research, hypothesis testing plays a crucial role in determining the validity of assumptions and drawing meaningful conclusions. By using statistical methods, researchers can evaluate hypotheses and make informed decisions based on the results. There are several types of hypothesis testing techniques commonly employed in market research. Let's explore them in detail:
1. One-Sample T-Test:
This type of hypothesis testing is used when comparing a sample mean to a known population mean. For instance, a market researcher may conduct a one-sample t-test to determine if the average age of their target customers is significantly different from the national average. By collecting a sample of data and performing the statistical analysis, they can make conclusions about the population as a whole.
2. independent Samples T-test :
When comparing the means of two independent groups, an independent samples t-test is the go-to method. For example, a market researcher may want to compare the average satisfaction levels of customers who purchased a product before and after a recent marketing campaign. By collecting data from both groups and conducting an independent samples t-test, they can determine if the campaign had a significant impact on customer satisfaction.
3. paired Samples T-test :
This type of hypothesis testing is similar to the independent samples t-test but is used when comparing means of two related groups. For instance, a market researcher may want to assess if there is a significant difference in customer ratings before and after a product upgrade. By collecting data from the same set of customers before and after the upgrade and performing a paired samples t-test, they can evaluate the impact of the upgrade on customer satisfaction .
4. chi-Square test :
The chi-square test is used when analyzing categorical data to determine if there is a significant association between two variables. For example, a market researcher may want to investigate if there is a relationship between gender and product preferences. By collecting data and conducting a chi-square test , they can assess if there is a significant difference in product preferences based on gender.
Tips for Effective Hypothesis Testing:
- Clearly define your research question and hypothesis before conducting any analysis. This will help guide your data collection and ensure you are testing the right variables.
- Ensure your sample size is appropriate for the type of hypothesis testing you are performing. inadequate sample sizes can lead to unreliable results.
- Choose the appropriate statistical test based on the nature of your data and research question. Using the wrong test may yield inaccurate conclusions.
- Take into account any potential confounding variables that may influence your results . Controlling for these variables will improve the validity of your hypothesis testing.
A market researcher wants to determine if offering a discount on a product will lead to increased sales. They randomly divide their customers into two groups : one group receives a discount, and the other group does not. After a specified period, they compare the sales data between the two groups using an independent samples t-test. The results show a significant difference in sales, indicating that offering a discount indeed leads to increased sales.
In conclusion, hypothesis testing in market research is a valuable tool for drawing meaningful insights and making data-driven decisions . By understanding the different types of hypothesis testing techniques and following best practices, researchers can ensure their findings are reliable and actionable.
Types of Hypothesis Testing in Market Research - Hypothesis testing: Hypothesis Testing in Market Research Methodology: A Beginner's Guide
1. Define your null and alternative hypotheses : The first step in conducting a hypothesis test is to clearly define your null and alternative hypotheses. The null hypothesis represents the status quo or the assumption that there is no significant difference or relationship between variables. On the other hand, the alternative hypothesis suggests that there is indeed a significant difference or relationship. For example, in a market research study , the null hypothesis could be that there is no difference in customer satisfaction between two different product packaging designs, while the alternative hypothesis would state that there is a significant difference.
2. Choose the appropriate test statistic: The next step is to determine the appropriate test statistic to use for your hypothesis test. This depends on the nature of your data and the type of hypothesis you are testing . For example, if you are comparing means between two groups , you might use a t-test, while for comparing proportions, a chi-square test could be more appropriate. It is crucial to select the right test statistic to ensure accurate results.
3. Set the significance level: The significance level, often denoted as (alpha), determines the threshold at which you will reject the null hypothesis. Commonly used significance levels are 0.05 and 0.01, indicating a 5% and 1% chance of rejecting the null hypothesis incorrectly, respectively. Choosing the significance level should be based on the importance of the decision and the potential consequences of making a Type I error (rejecting the null hypothesis when it is true) or a Type II error (failing to reject the null hypothesis when it is false).
4. collect and analyze data : With the hypotheses defined, test statistic selected, and significance level set, it's time to collect the necessary data and analyze it using the chosen statistical test. This step involves conducting the statistical calculations and interpreting the results to determine whether the null hypothesis should be rejected or not. For instance, if the calculated p-value is less than the significance level, we reject the null hypothesis in favor of the alternative hypothesis.
5. draw conclusions and make recommendations : Once the results are obtained, it is essential to draw conclusions based on the findings of the hypothesis test. If the null hypothesis is rejected, it suggests that the alternative hypothesis is supported, indicating a significant difference or relationship between variables. These conclusions can then be used to make informed decisions or recommendations in the context of the market research study.
- Ensure that your sample size is large enough to provide reliable results. A small sample size may lead to inconclusive or unreliable findings.
- Take into account any potential confounding variables or biases that may influence the results of your hypothesis test. Proper experimental design and control measures can help mitigate these issues.
- Consider conducting a power analysis to determine the required sample size for your study. This will help ensure that you have sufficient statistical power to detect meaningful effects.
Suppose a market researcher wants to investigate whether a new advertising campaign has a significant impact on brand awareness . The null hypothesis would state that there is no significant difference in brand awareness before and after the campaign, while the alternative hypothesis would suggest that the campaign does have a significant impact. By collecting data on brand awareness before and after the campaign and conducting a hypothesis test, the researcher can determine the effectiveness of the advertising campaign .
In conclusion, conducting a hypothesis test is a crucial step in market research methodology. By following a step-by-step guide , researchers can ensure that their hypothesis tests are conducted accurately and produce meaningful results. By understanding the process and considering important factors such as hypotheses, test statistics, significance levels, and sample sizes, researchers can make informed decisions and recommendations based on their findings.
Step by Step Guide - Hypothesis testing: Hypothesis Testing in Market Research Methodology: A Beginner's Guide
Once you have conducted a hypothesis test, it is crucial to interpret the results accurately. This step is essential in determining whether to accept or reject the null hypothesis and draw meaningful conclusions from your research. In this section, we will discuss some key aspects to consider when interpreting the results of a hypothesis test.
1. Statistical Significance:
One of the primary factors to consider is the statistical significance of your findings. Statistical significance refers to the likelihood that the observed results are not due to chance. Typically, researchers use a significance level (alpha level) to determine whether the p-value obtained from the test is small enough to reject the null hypothesis. For instance, if you set your significance level at 0.05 and obtain a p-value of 0.03, you can conclude that the results are statistically significant and reject the null hypothesis.
Example: Suppose you conducted a hypothesis test to determine whether a new marketing campaign increased sales. Your null hypothesis states that the campaign had no effect, while the alternative hypothesis suggests that the campaign did have an impact. If your p-value is less than your significance level, say 0.05, you can reject the null hypothesis, indicating that the campaign did indeed have an effect on sales.
2. Effect Size:
While statistical significance is essential , it is equally important to consider the effect size. Effect size measures the magnitude of the difference or relationship between variables in your study. It provides a more comprehensive understanding of the practical significance of your findings. By examining the effect size, you can determine the practical relevance of your results.
Example: Let's say you conducted a hypothesis test to compare the average satisfaction levels of two different customer service approaches. While your test may indicate a statistically significant difference, the effect size shows that the difference is minimal. In this case, the statistical significance may not be practically meaningful, and you may need to consider alternative strategies to improve customer satisfaction .
3. Confidence Intervals:
Interpreting the results of a hypothesis test can be enhanced by considering confidence intervals. A confidence interval provides a range of values within which the true population parameter is likely to fall. It adds a level of precision to your results and helps assess the uncertainty associated with your findings.
Example: Suppose you conducted a hypothesis test to determine whether a new product launch increased customer loyalty . While your test may show a statistically significant increase, the confidence interval indicates that the true increase in customer loyalty could range from 5% to 15%. This broader range helps you understand the potential impact of the new product on customer loyalty more accurately.
- Always consider the context of the research question and the specific goals of your study when interpreting hypothesis test results.
- Remember that statistical significance does not always guarantee practical significance. Always consider the effect size to assess the importance of your findings.
- Confidence intervals provide valuable information about the precision and uncertainty of your results. Take them into account when drawing conclusions .
In a recent market research study, a company wanted to determine if a new packaging design for their product would lead to increased sales. After conducting a hypothesis test, the results showed a statistically significant increase in sales with the new packaging. However, when analyzing the effect size, it was found that the increase was only 1%. Although statistically significant, the effect size was deemed too small to justify the costs associated with implementing the new packaging design. Therefore, the company decided to stick with the current packaging and explore other strategies to boost sales .
Interpreting the results of a hypothesis test requires careful consideration of statistical significance, effect size, and confidence intervals. By understanding these key elements , researchers can draw meaningful conclusions and make informed decisions based on their findings.
Interpreting the Results of a Hypothesis Test - Hypothesis testing: Hypothesis Testing in Market Research Methodology: A Beginner's Guide
Hypothesis testing is a fundamental aspect of market research methodology that helps researchers make informed decisions based on data analysis. However, it is not without its pitfalls and challenges. In this section, we will explore some of the common mistakes that researchers often encounter during hypothesis testing and provide tips to overcome them.
1. Insufficient sample size: One of the most prevalent challenges in hypothesis testing is having an inadequate sample size. A small sample may not accurately represent the target population, leading to biased results. For example, if a market research study aims to understand consumer preferences for a new product, a sample size of only 10 participants may not provide a comprehensive understanding of the entire consumer base. To mitigate this challenge, researchers should ensure that their sample size is statistically significant and representative of the population they are studying.
2. Type I and Type II errors: Another common pitfall in hypothesis testing is the occurrence of Type I and Type II errors. Type I error, also known as a false positive, happens when a researcher rejects a true null hypothesis. On the other hand, Type II error, or false negative, occurs when a researcher fails to reject a false null hypothesis. Both types of errors can lead to incorrect conclusions and misinterpretations of data. To minimize the risk of these errors, researchers must carefully choose the significance level (alpha) and power of their statistical tests .
3. Violation of assumptions: Hypothesis tests are based on certain assumptions, and violating these assumptions can compromise the validity of the results. For instance, the t-test assumes that the data is normally distributed, while the ANOVA assumes homogeneity of variances. If these assumptions are not met, the hypothesis test results may be inaccurate. To avoid this pitfall, researchers should assess the assumptions of their chosen statistical tests and consider alternative methods if the assumptions are violated.
4. Multiple hypothesis testing: Market research often involves testing multiple hypotheses simultaneously , which can increase the likelihood of false positives. When conducting multiple tests, the probability of at least one significant result due to chance alone also increases. This is known as the problem of multiple comparisons . To address this challenge, researchers can adjust the significance level using Bonferroni correction or other methods to maintain the overall desired level of statistical significance.
5. confirmation bias : Confirmation bias occurs when researchers unconsciously favor information that confirms their preconceived notions or hypotheses. This bias can lead to cherry-picking data or interpreting results in a way that supports the desired outcome. To overcome confirmation bias, it is crucial for researchers to approach hypothesis testing with an open mind and remain objective throughout the entire research process.
Case Study: A market research team conducted a study to determine whether a new advertising campaign had a significant impact on brand awareness. They collected data from a sample of 500 participants and performed a hypothesis test. The results showed a p-value of 0.02, indicating statistical significance. However, upon further investigation, it was discovered that the sample was primarily composed of individuals already familiar with the brand. This biased sample led to an overestimation of the advertising campaign's impact on brand awareness. By acknowledging the pitfall of insufficient sample size and ensuring a more representative sample, the researchers could have obtained more accurate results.
In conclusion, hypothesis testing in market research methodology is not without its challenges. However, by being aware of common pitfalls and implementing appropriate strategies, researchers can improve the validity and reliability of their findings. It is essential to carefully consider sample size, minimize Type I and Type II errors, adhere to assumptions, address multiple hypothesis testing, and remain vigilant against confirmation bias. By doing so, researchers can make more informed decisions based on robust data analysis .
Common Pitfalls and Challenges in Hypothesis Testing - Hypothesis testing: Hypothesis Testing in Market Research Methodology: A Beginner's Guide
1. Clearly define your research objectives: Before conducting any hypothesis testing in market research, it is crucial to have a clear understanding of your research objectives. Clearly define what you aim to achieve and the specific questions you want to answer through your research. This will help you formulate relevant hypotheses and ensure that your testing is focused and meaningful.
2. Use a sample size calculator: determining the appropriate sample size is essential to ensure the validity and reliability of your hypothesis testing results. By using a sample size calculator, you can determine the sample size needed to detect a specific effect size with a desired level of statistical power. This will help you optimize your resources and avoid wasting time and effort on an inadequate sample size.
3. Develop testable hypotheses: A hypothesis should be specific, measurable, and testable. Avoid vague or general statements that cannot be empirically tested. For example, instead of stating "Customers prefer our product," a testable hypothesis could be "Customers who have used our product rate it higher than competing products on a satisfaction scale."
4. Choose the appropriate statistical test: Depending on the nature of your data and research objectives, different statistical tests may be required. It is important to select the appropriate test that matches your research design and the type of data you have collected. For example, if you want to compare the means of two independent groups, a t-test would be suitable, whereas an ANOVA would be appropriate for comparing means across multiple groups.
5. Set the significance level and interpret results accordingly: The significance level, often denoted as alpha (), determines the threshold at which you reject or fail to reject the null hypothesis. Commonly used levels include 0.05 and 0.01. It is crucial to set your significance level before conducting the test and interpret the results accordingly. Failing to do so may lead to biased interpretations or cherry-picking results.
6. Consider potential confounding variables: Confounding variables are factors that may affect the relationship between the independent and dependent variables, leading to inaccurate conclusions. It is important to identify and control for potential confounding variables to ensure the validity of your hypothesis testing. For instance, if you are testing the impact of a marketing campaign on sales , you should consider factors like seasonality or competitor activities that may influence sales independently of your campaign.
7. Document your methodology and assumptions: Transparency is key in market research. Documenting your methodology and assumptions allows others to replicate or critique your study. Clearly state the statistical tests used, sample size, significance level, and any assumptions made during the analysis. This documentation will enhance the credibility of your findings and facilitate future research.
8. Validate your findings with additional research: Hypothesis testing is just one step in the market research process . To ensure the reliability and generalizability of your findings, consider conducting additional research using different methods or in different contexts. This will help validate your initial hypotheses and provide a more comprehensive understanding of the phenomenon under study.
In conclusion, effective hypothesis testing is crucial for obtaining meaningful insights in market research . By following these best practices, you can ensure that your testing is focused, valid, and reliable. Remember to clearly define your research objectives, use a sample size calculator, develop testable hypotheses, choose the appropriate statistical test, set the significance level, consider potential confounding variables, document your methodology and assumptions, and validate your findings with additional research. By adhering to these practices, you can enhance the quality and impact of your market research endeavors.
Best Practices for Effective Hypothesis Testing in Market Research - Hypothesis testing: Hypothesis Testing in Market Research Methodology: A Beginner's Guide
In this blog, we have explored the concept of hypothesis testing in market research and its significance in informing data-driven decision-making . By formulating and testing hypotheses , researchers can gain valuable insights into consumer behavior, market trends, and the effectiveness of marketing strategies . Let's summarize the key points discussed throughout this guide:
1. Hypothesis testing provides a structured approach: By following a systematic process, researchers can ensure that their findings are based on reliable evidence rather than mere speculation. This approach involves formulating a clear research question, developing a hypothesis, collecting data, analyzing the results, and drawing conclusions.
2. Examples of hypothesis testing in market research: To illustrate the practical application of hypothesis testing, let's consider a hypothetical scenario. A company wants to determine if changing the packaging design of their product will increase sales. The null hypothesis could be that there is no significant difference in sales between the old and new packaging, while the alternative hypothesis suggests that the new packaging leads to higher sales. By conducting a controlled experiment and analyzing the data using appropriate statistical tests, the company can determine if their hypothesis is supported or rejected.
3. Tips for effective hypothesis testing: When conducting hypothesis testing in market research, it is crucial to consider the following tips:
A. Clearly define the research question and hypotheses: The research question should be specific and focused, while the hypotheses should be testable and mutually exclusive.
B. Use appropriate statistical methods: Selecting the right statistical tests ensures accurate analysis and interpretation of the data. Consult with a statistician or use software tools that can guide you in the selection process.
C. Consider sample size and representativeness : A larger sample size generally leads to more reliable results. Additionally, ensure that your sample is representative of the target population to avoid biased conclusions.
D. Beware of Type I and Type II errors: Type I error occurs when a true null hypothesis is rejected, while Type II error happens when a false null hypothesis is accepted. Understanding these errors helps researchers interpret their findings correctly.
4. case studies showcasing hypothesis testing in market research: Real-world examples can provide valuable insights into how hypothesis testing is applied in different industries. For instance, a retail company might test the hypothesis that offering personalized discounts based on customer preferences will lead to increased customer loyalty and repeat purchases . By analyzing customer data and conducting surveys, the company can evaluate the effectiveness of their personalized discount strategy and make data-driven decisions .
In conclusion, hypothesis testing is a powerful tool in market research that enables researchers to make evidence-based decisions. By following a structured approach, using appropriate statistical methods, and considering key factors such as sample size and representativeness, researchers can harness the power of hypothesis testing to gain valuable insights into consumer behavior and market trends.
Harnessing the Power of Hypothesis Testing in Market Research - Hypothesis testing: Hypothesis Testing in Market Research Methodology: A Beginner's Guide
Read Other Blogs
Visual Basic for Applications (VBA) is a powerful scripting language that operates within Excel,...
Visual branding and website design are inextricably linked in the digital age. A company's online...
Venture capital represents a vital force in the economic landscape of any country, acting as a...
Embarking on the journey from debt to solvency is a transformative process that businesses of all...
Dialectical behavior therapy (DBT) is a type of cognitive-behavioral therapy that was originally...
In the pulsating world of mobile commerce, revenue models are the engines driving profitability....
Social media monitoring is an essential practice for any brand that wants to stay ahead of the...
Understanding your customers is the cornerstone of any successful business strategy. It's the...
In the quest for productivity and work-life balance, the concept of segmenting one's day into...
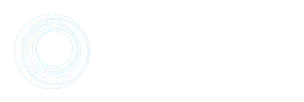
Expert Advice on Developing a Hypothesis for Marketing Experimentation
- Conversion Rate Optimization
Simbar Dube
Every marketing experimentation process has to have a solid hypothesis.
That’s a must – unless you want to be roaming in the dark and heading towards a dead-end in your experimentation program.
Hypothesizing is the second phase of our SHIP optimization process here at Invesp.
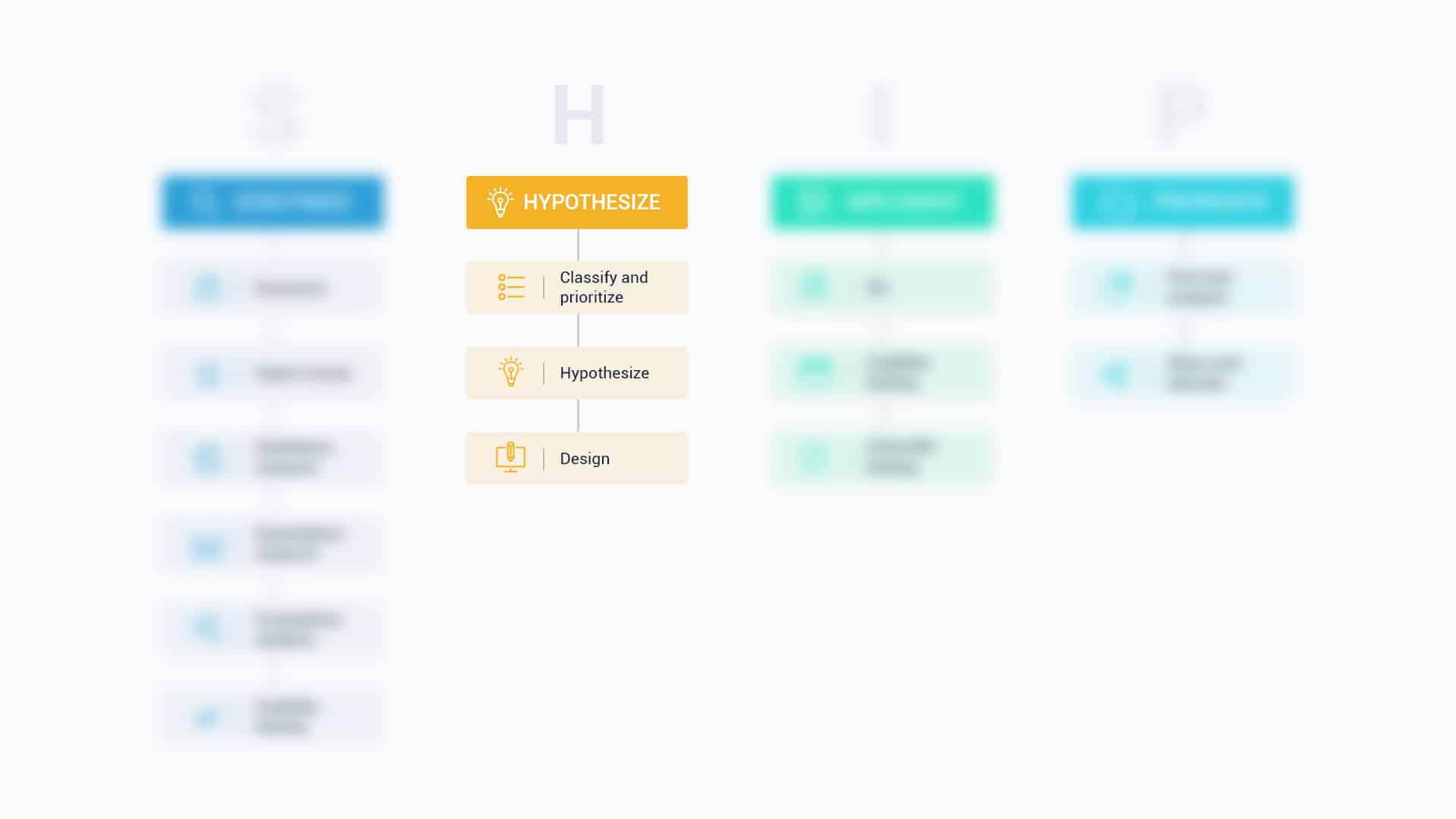
It comes after we have completed the research phase.
This is an indication that we don’t just pull a hypothesis out of thin air. We always make sure that it is based on research data.
But having a research-backed hypothesis doesn’t mean that the hypothesis will always be correct. In fact, tons of hypotheses bear inconclusive results or get disproved.
The main idea of having a hypothesis in marketing experimentation is to help you gain insights – regardless of the testing outcome.
By the time you finish reading this article, you’ll know:
- The essential tips on what to do when crafting a hypothesis for marketing experiments
- How a marketing experiment hypothesis works
How experts develop a solid hypothesis
The basics: marketing experimentation hypothesis.
A hypothesis is a research-based statement that aims to explain an observed trend and create a solution that will improve the result. This statement is an educated, testable prediction about what will happen.
It has to be stated in declarative form and not as a question.
“ If we add magnification info, product video and making virtual mirror buttons, will that improve engagement? ” is not declarative, but “ Improving the experience of product pages by adding magnification info, product video and making virtual mirror buttons will increase engagement ” is.
Here’s a quick example of how a hypothesis should be phrased:
- Replacing ___ with __ will increase [conversion goal] by [%], because:
- Removing ___ and __ will decrease [conversion goal] by [%], because:
- Changing ___ into __ will not affect [conversion goal], because:
- Improving ___ by ___will increase [conversion goal], because:
As you can see from the above sentences, a good hypothesis is written in clear and simple language. Reading your hypothesis should tell your team members exactly what you thought was going to happen in an experiment.
Another important element of a good hypothesis is that it defines the variables in easy-to-measure terms, like who the participants are, what changes during the testing, and what the effect of the changes will be:
Example : Let’s say this is our hypothesis:
Displaying full look items on every “continue shopping & view your bag” pop-up and highlighting the value of having a full look will improve the visibility of a full look, encourage visitors to add multiple items from the same look and that will increase the average order value, quantity with cross-selling by 3% .
Who are the participants :
Visitors.
What changes during the testing :
Displaying full look items on every “continue shopping & view your bag” pop-up and highlighting the value of having a full look…
What the effect of the changes will be:
Will improve the visibility of a full look, encourage visitors to add multiple items from the same look and that will increase the average order value, quantity with cross-selling by 3% .
Don’t bite off more than you can chew! Answering some scientific questions can involve more than one experiment, each with its own hypothesis. so, you have to make sure your hypothesis is a specific statement relating to a single experiment.
How a Marketing Experimentation Hypothesis Works
Assuming that you have done conversion research and you have identified a list of issues ( UX or conversion-related problems) and potential revenue opportunities on the site. The next thing you’d want to do is to prioritize the issues and determine which issues will most impact the bottom line.
Having ranked the issues you need to test them to determine which solution works best. At this point, you don’t have a clear solution for the problems identified. So, to get better results and avoid wasting traffic on poor test designs, you need to make sure that your testing plan is guided.
This is where a hypothesis comes into play.
For each and every problem you’re aiming to address, you need to craft a hypothesis for it – unless the problem is a technical issue that can be solved right away without the need to hypothesize or test.
One important thing you should note about an experimentation hypothesis is that it can be implemented in different ways.
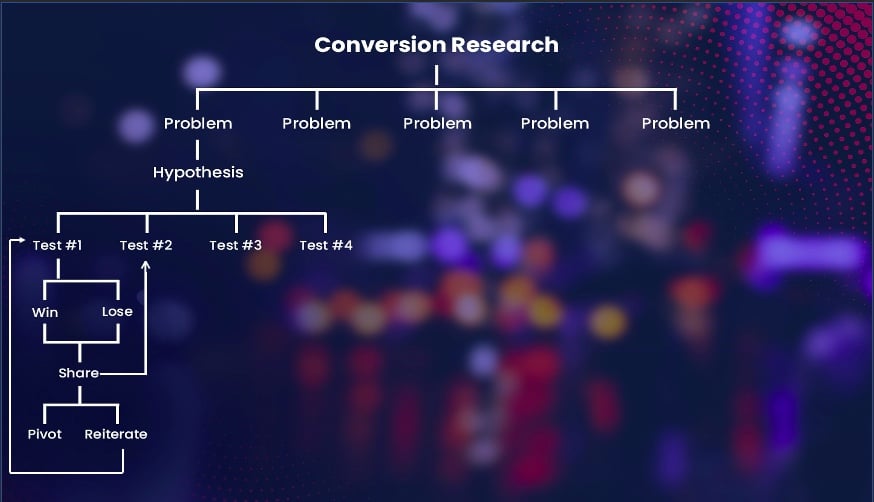
This means that one hypothesis can have four or five different tests as illustrated in the image above. Khalid Saleh , the Invesp CEO, explains:
“There are several ways that can be used to support one single hypothesis. Each and every way is a possible test scenario. And that means you also have to prioritize the test design you want to start with. Ultimately the name of the game is you want to find the idea that has the biggest possible impact on the bottom line with the least amount of effort. We use almost 18 different metrics to score all of those.”
In one of the recent tests we launched after watching video recordings, viewing heatmaps, and conducting expert reviews, we noticed that:
- Visitors were scrolling to the bottom of the page to fill out a calculator so as to get a free diet plan.
- Brand is missing
- Too many free diet plans – and this made it hard for visitors to choose and understand.
- No value proposition on the page
- The copy didn’t mention the benefits of the paid program
- There was no clear CTA for the next action
To help you understand, let’s have a look at how the original page looked like before we worked on it:
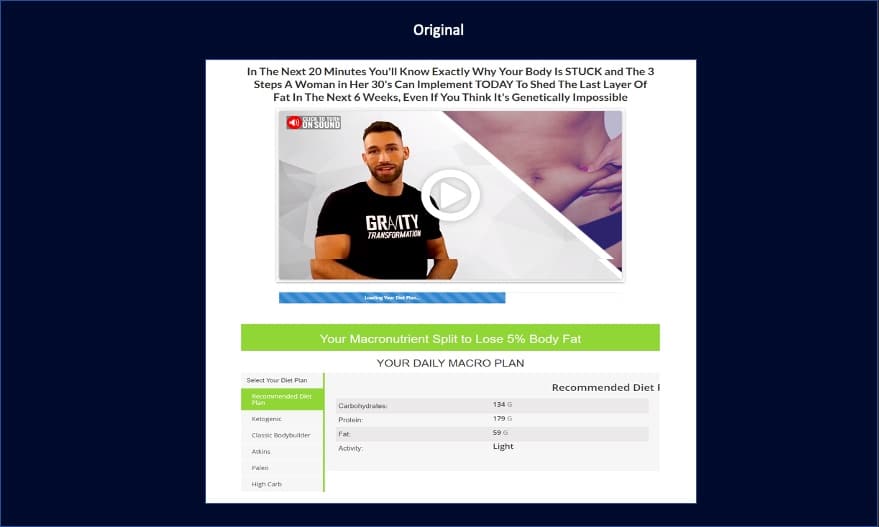
So our aim was to make the shopping experience seamless for visitors, make the page more appealing and not confusing. In order to do that, here is how we phrased the hypothesis for the page above:
Improving the experience of optin landing pages by making the free offer accessible above the fold and highlighting the next action with a clear CTA and will increase the engagement on the offer and increase the conversion rate by 1%.
For this particular hypothesis, we had two design variations aligned to it:
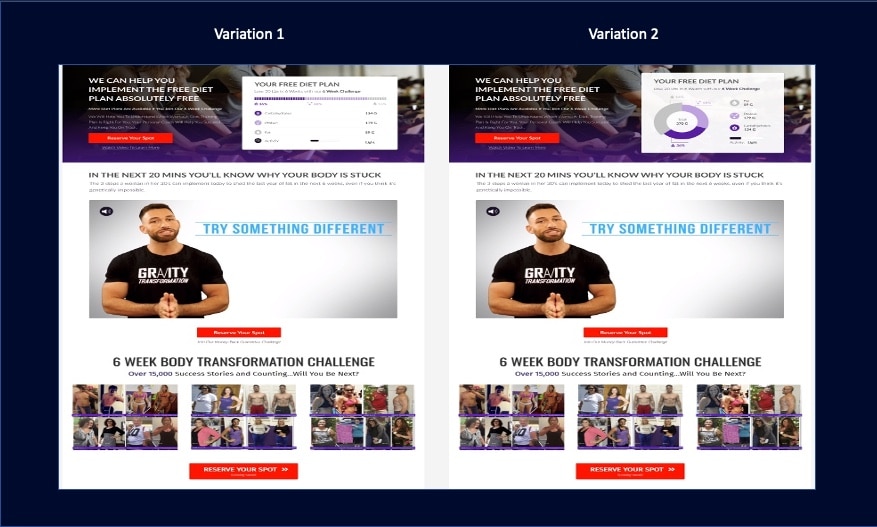
The two above designs are different, but they are aligned to one hypothesis. This goes on to show how one hypothesis can be implemented in different ways. Looking at the two variations above – which one do you think won?
Yes, you’re right, V2 was the winner.
Considering that there are many ways you can implement one hypothesis, so when you launch a test and it fails, it doesn’t necessarily mean that the hypothesis was wrong. Khalid adds:
“A single failure of a test doesn’t mean that the hypothesis is incorrect. Nine times out of ten it’s because of the way you’ve implemented the hypothesis. Look at the way you’ve coded and look at the copy you’ve used – you are more likely going to find something wrong with it. Always be open.”
So there are three things you should keep in mind when it comes to marketing experimentation hypotheses:
- It takes a while for this hypothesis to really fully test it.
- A single failure doesn’t necessarily mean that the hypothesis is incorrect.
- Whether a hypothesis is proved or disproved, you can still learn something about your users.
I know it’s never easy to develop a hypothesis that informs future testing – I mean it takes a lot of intense research behind the scenes, and tons of ideas to begin with. So, I reached out to six CRO experts for tips and advice to help you understand more about developing a solid hypothesis and what to include in it.
Maurice says that a solid hypothesis should have not more than one goal:
Maurice Beerthuyzen – CRO/CXO Lead at ClickValue “Creating a hypothesis doesn’t begin at the hypothesis itself. It starts with research. What do you notice in your data, customer surveys, and other sources? Do you understand what happens on your website? When you notice an opportunity it is tempting to base one single A/B test on one hypothesis. Create hypothesis A and run a single test, and then move forward to the next test. With another hypothesis. But it is very rare that you solve your problem with only one hypothesis. Often a test provides several other questions. Questions which you can solve with running other tests. But based on that same hypothesis! We should not come up with a new hypothesis for every test. Another mistake that often happens is that we fill the hypothesis with multiple goals. Then we expect that the hypothesis will work on conversion rate, average order value, and/or Click Through Ratio. Of course, this is possible, but when you run your test, your hypothesis can only have one goal at once. And what if you have two goals? Just split the hypothesis then create a secondary hypothesis for your second goal. Every test has one primary goal. What if you find a winner on your secondary hypothesis? Rerun the test with the second hypothesis as the primary one.”
Jon believes that a strong hypothesis is built upon three pillars:
Jon MacDonald – President and Founder of The Good Respond to an established challenge – The challenge must have a strong background based on data, and the background should state an established challenge that the test is looking to address. Example: “Sign up form lacks proof of value, incorrectly assuming if users are on the page, they already want the product.” Propose a specific solution – What is the one, the single thing that is believed will address the stated challenge? Example: “Adding an image of the dashboard as a background to the signup form…”. State the assumed impact – The assumed impact should reference one specific, measurable optimization goal that was established prior to forming a hypothesis. Example: “…will increase signups.” So, if your hypothesis doesn’t have a specific, measurable goal like “will increase signups,” you’re not really stating a test hypothesis!”
Matt uses his own hypothesis builder to collate important data points into a single hypothesis.
Matt Beischel – Founder of Corvus CRO Like Jon, Matt also breaks down his hypothesis writing process into three sections. Unlike Jon, Matt sections are: Comprehension Response Outcome I set it up so that the names neatly match the “CRO.” It’s a sort of “mad-libs” style fill-in-the-blank where each input is an important piece of information for building out a robust hypothesis. I consider these the minimum required data points for a good hypothesis; if you can’t completely fill out the form, then you don’t have a good hypothesis. Here’s a breakdown of each data point: Comprehension – Identifying something that can be improved upon Problem: “What is a problem we have?” Observation Method: “How did we identify the problem?” Response – Change that can cause improvement Variation: “What change do we think could solve the problem?” Location: “Where should the change occur?” Scope: “What are the conditions for the change?” Audience: “Who should the change affect?” Outcome – Measurable result of the change that determines the success Behavior Change : “What change in behavior are we trying to affect?” Primary KPI: “What is the important metric that determines business impact?” Secondary KPIs: “Other metrics that will help reinforce/refute the Primary KPI” Something else to consider is that I have a “user first” approach to formulating hypotheses. My process above is always considered within the context of how it would first benefit the user. Now, I do feel that a successful experiment should satisfy the needs of BOTH users and businesses, but always be in favor of the user. Notice that “Behavior Change” is the first thing listed in Outcome, not primary business KPI. Sure, at the end of the day you are working for the business’s best interests (both strategically and financially), but placing the user first will better inform your decision making and prioritization; there’s a reason that things like personas, user stories, surveys, session replays, reviews, etc. exist after all. A business-first ideology is how you end up with dark patterns and damaging brand credibility.”
One of the many mistakes that CROs make when writing a hypothesis is that they are focused on wins and not on insights. Shiva advises against this mindset:
Shiva Manjunath – Marketing Manager and CRO at Gartner “Test to learn, not test to win. It’s a very simple reframe of hypotheses but can have a magnitude of difference. Here’s an example: Test to Win Hypothesis: If I put a product video in the middle of the product page, I will improve add to cart rates and improve CVR. Test to Learn Hypothesis: If I put a product video on the product page, there will be high engagement with the video and it will positively influence traffic What you’re doing is framing your hypothesis, and test, in a particular way to learn as much as you can. That is where you gain marketing insights. The more you run ‘marketing insight’ tests, the more you will win. Why? The more you compound marketing insight learnings, your win velocity will start to increase as a proxy of the learnings you’ve achieved. Then, you’ll have a higher chance of winning in your tests – and the more you’ll be able to drive business results.”
Lorenzo says it’s okay to focus on achieving a certain result as long as you are also getting an answer to: “Why is this event happening or not happening?”
Lorenzo Carreri – CRO Consultant “When I come up with a hypothesis for a new or iterative experiment, I always try to find an answer to a question. It could be something related to a problem people have or an opportunity to achieve a result or a way to learn something. The main question I want to answer is “Why is this event happening or not happening?” The question is driven by data, both qualitative and quantitative. The structure I use for stating my hypothesis is: From [data source], I noticed [this problem/opportunity] among [this audience of users] on [this page or multiple pages]. So I believe that by [offering this experiment solution], [this KPI] will [increase/decrease/stay the same].
Jakub Linowski says that hypotheses are meant to hold researchers accountable:
Jakub Linowski – Chief Editor of GoodUI “They do this by making your change and prediction more explicit. A typical hypothesis may be expressed as: If we change (X), then it will have some measurable effect (A). Unfortunately, this oversimplified format can also become a heavy burden to your experiment design with its extreme reductionism. However you decide to format your hypotheses, here are three suggestions for more flexibility to avoid limiting yourself. One Or More Changes To break out of the first limitation, we have to admit that our experiments may contain a single or multiple changes. Whereas the classic hypothesis encourages a single change or isolated variable, it’s not the only way we can run experiments. In the real world, it’s quite normal to see multiple design changes inside a single variation. One valid reason for doing this is when wishing to optimize a section of a website while aiming for a greater effect. As more positive changes compound together, there are times when teams decide to run bigger experiments. An experiment design (along with your hypotheses) therefore should allow for both single or multiple changes. One Or More Metrics A second limitation of many hypotheses is that they often ask us to only make a single prediction at a time. There are times when we might like to make multiple guesses or predictions to a set of metrics. A simple example of this might be a trade-off experiment with a guess of increased sales but decreased trial signups. Being able to express single or multiple metrics in our experimental designs should therefore be possible. Estimates, Directional Predictions, Or Unknowns Finally, traditional hypotheses also tend to force very simple directional predictions by asking us to guess whether something will increase or decrease. In reality, however, the fidelity of predictions can be higher or lower. On one hand, I’ve seen and made experiment estimations that contain specific numbers from prior data (ex: increase sales by 14%). While at other times it should also be acceptable to admit the unknown and leave the prediction blank. One example of this is when we are testing a completely novel idea without any prior data in a highly exploratory type of experiment. In such cases, it might be dishonest to make any sort of predictions and we should allow ourselves to express the unknown comfortably.”
Conclusion
So there you have it! Before you jump on launching a test, start by making sure that your hypothesis is solid and backed by research. Ask yourself the questions below when crafting a hypothesis for marketing experimentation:
- Is the hypothesis backed by research?
- Can the hypothesis be tested?
- Does the hypothesis provide insights?
- Does the hypothesis set the expectation that there will be an explanation behind the results of whatever you’re testing?
Don’t worry! Hypothesizing may seem like a very complicated process, but it’s not complicated in practice especially when you have done proper research.
If you enjoyed reading this article and you’d love to get the best CRO content – delivered by the best experts in the industry – straight to your inbox, every week. Please subscribe here .
Share This Article
Join 25,000+ marketing professionals.
Subscribe to Invesp’s blog feed for future articles delivered to receive weekly updates by email.
Discover Similar Topics
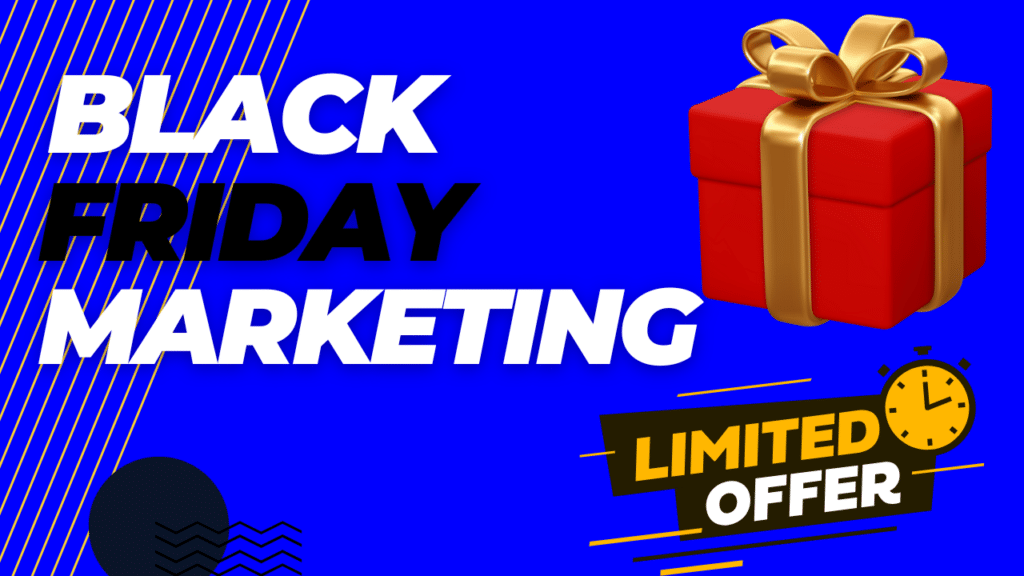
10 Successful Black Friday Marketing Strategies
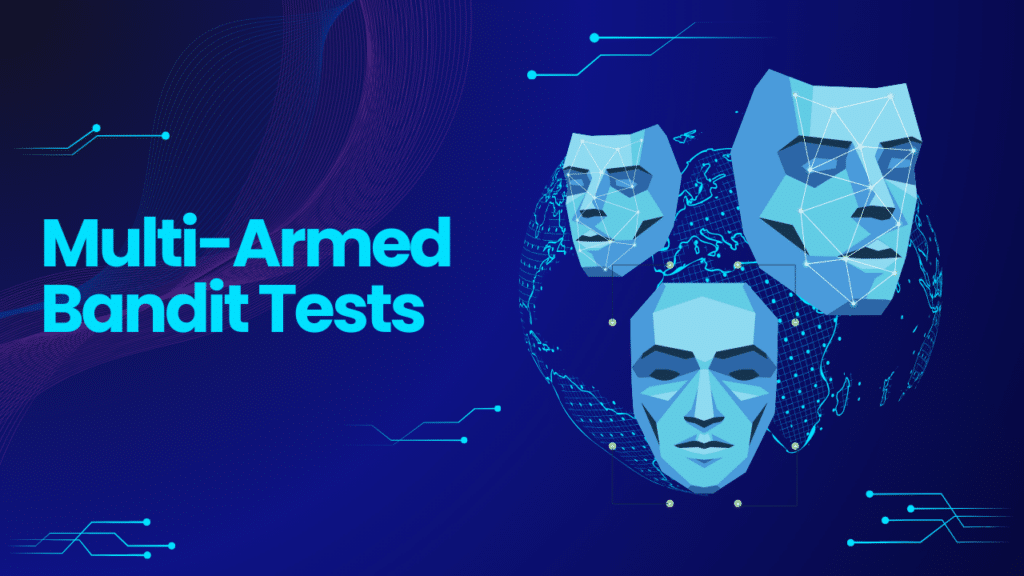
The Power of Multi-Armed Bandit Tests for Marketers
- A/B Testing
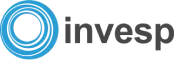
Our Services
- Conversion Optimization Training
- Conversion Rate Optimization Professional Services
- Landing Page Optimization
- Conversion Rate Audit
- Design for Growth
- Conversion Research & Discovery
- End to End Digital Optimization
By Industry
- E-commerce CRO Services
- Lead Generation CRO Services
- SaaS CRO Services
- Startup CRO Program
- Case Studies
- Privacy Policy
- © 2006-2020 All rights reserved. Invesp
Subscribe with us
- US office: Chicago, IL
- European office: Istanbul, Turkey
- +1.248.270.3325
- [email protected]
- Conversion Rate Optimization Services
- © 2006-2023 All rights reserved. Invesp
- Popular Topics
- Business & Growth
- Copywriting
- Infographics
- Landing Pages
- Sales & Marketing
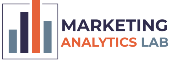
Marketing Analytics Lab

Hypothesis Testing in Marketing Research
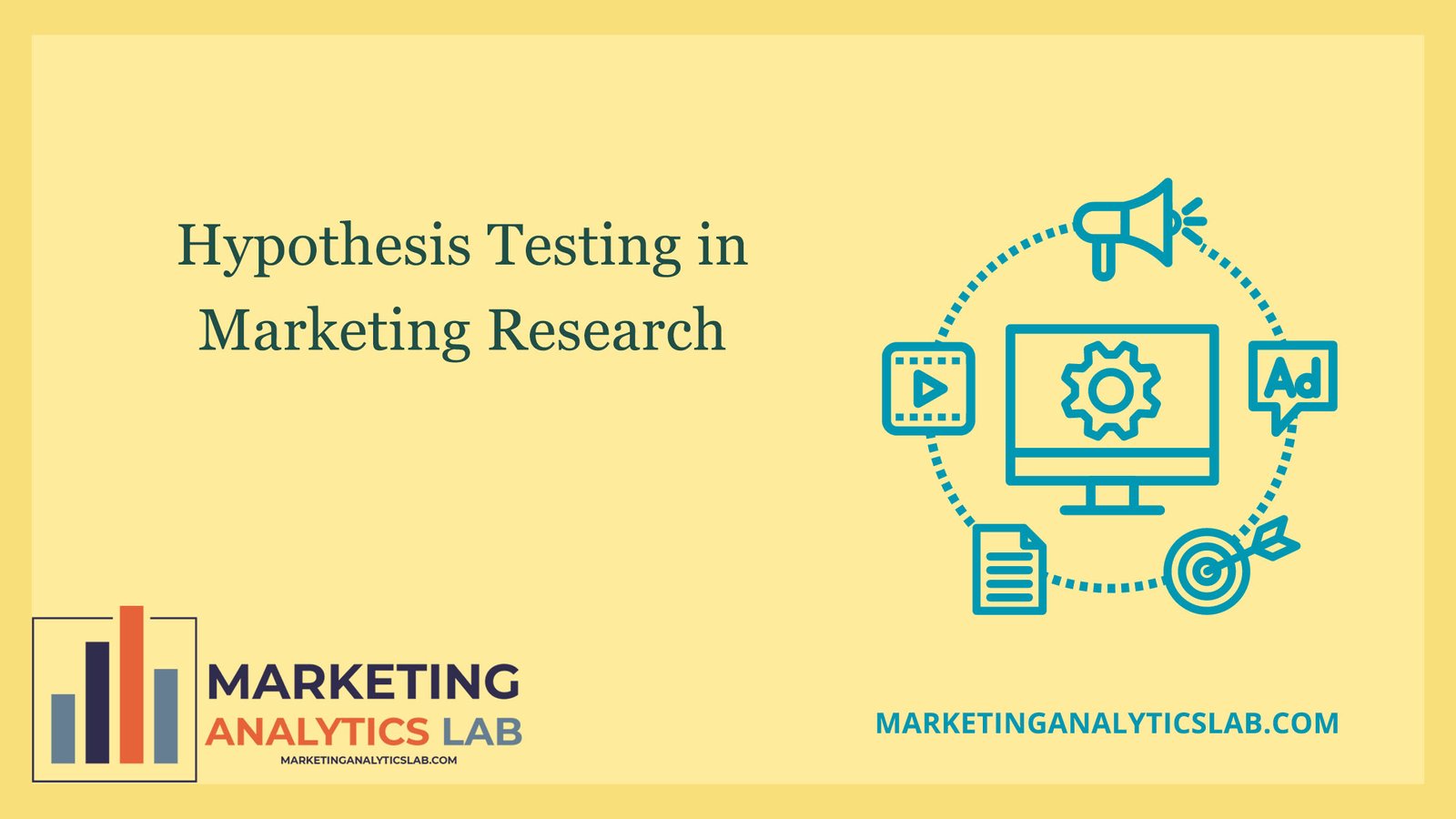
Hypothesis testing is a fundamental statistical method used in marketing research to make inferences about a population based on sample data. It helps researchers and marketers determine whether there is enough evidence to support a specific claim or hypothesis about consumer behavior, market trends, or the effectiveness of marketing strategies. This article explores the principles of hypothesis testing, its application in marketing research, and the key concepts and techniques involved.
1. What is Hypothesis Testing?
Overview : Hypothesis testing is a statistical procedure used to evaluate whether a hypothesis about a population parameter is supported by sample data. The process involves making a claim, testing it against observed data, and determining whether to accept or reject the claim based on statistical evidence.
Key Concepts :
- Null Hypothesis (H₀) : The null hypothesis represents the default assumption that there is no effect or difference. It is the hypothesis that researchers seek to test against.
- Alternative Hypothesis (H₁ or Ha) : The alternative hypothesis represents the claim that there is an effect or difference. It is what researchers aim to provide evidence for.
- Significance Level (α) : The significance level, often set at 0.05, is the probability of rejecting the null hypothesis when it is actually true. It defines the threshold for determining statistical significance.
- P-Value : The p-value is the probability of obtaining test results at least as extreme as the observed results, assuming the null hypothesis is true. A p-value less than the significance level indicates strong evidence against the null hypothesis.
- Test Statistic : The test statistic is a standardized value calculated from the sample data used to determine whether to reject the null hypothesis. Common test statistics include t-values, z-values, and F-values.
2. Steps in Hypothesis Testing
1. Formulate Hypotheses :
- Null Hypothesis (H₀) : State the default assumption, such as “There is no difference in customer satisfaction before and after implementing a new marketing campaign.”
- Alternative Hypothesis (H₁) : State the claim being tested, such as “There is a difference in customer satisfaction before and after implementing a new marketing campaign.”
2. Choose the Significance Level (α) :
- Typically set at 0.05, 0.01, or 0.10, depending on the research context and desired level of confidence.
3. Collect and Analyze Data :
- Gather data through surveys, experiments, or other methods and calculate the test statistic based on the sample data.
4. Determine the P-Value :
- Compare the p-value to the significance level to assess the strength of evidence against the null hypothesis.
5. Make a Decision :
- Reject H₀ : If the p-value is less than the significance level, reject the null hypothesis and accept the alternative hypothesis.
- Fail to Reject H₀ : If the p-value is greater than the significance level, fail to reject the null hypothesis.
6. Draw Conclusions :
- Interpret the results in the context of the research question and make recommendations based on the findings.
3. Applications in Marketing Research
A. evaluating marketing campaign effectiveness.
Overview : Hypothesis testing can assess whether a marketing campaign has significantly impacted consumer behavior or sales performance.
- Null Hypothesis (H₀) : “The new marketing campaign has no effect on sales.”
- Alternative Hypothesis (H₁) : “The new marketing campaign has a significant effect on sales.”
Application :
- Analyze pre- and post-campaign sales data to determine if there is a statistically significant increase in sales.
- Data-Driven Decisions : Make informed decisions about continuing, modifying, or discontinuing marketing campaigns based on statistical evidence.
b. Testing Product Preferences
Overview : Hypothesis testing can help understand consumer preferences and evaluate whether different product features or attributes influence purchasing decisions.
- Null Hypothesis (H₀) : “There is no difference in consumer preference between Product A and Product B.”
- Alternative Hypothesis (H₁) : “There is a significant difference in consumer preference between Product A and Product B.”
- Conduct surveys or experiments to compare consumer preferences and analyze the results to determine if there is a significant difference.
- Product Development : Use findings to guide product development and design strategies that align with consumer preferences.
c. Assessing Customer Satisfaction
Overview : Hypothesis testing can evaluate changes in customer satisfaction levels due to changes in products, services, or customer experience initiatives.
- Null Hypothesis (H₀) : “Customer satisfaction scores have not changed after implementing the new customer service strategy.”
- Alternative Hypothesis (H₁) : “Customer satisfaction scores have significantly changed after implementing the new customer service strategy.”
- Analyze customer satisfaction survey data before and after the implementation of the strategy to assess the impact.
- Improvement Strategies : Identify effective strategies for enhancing customer satisfaction and loyalty.
d. Market Segmentation Analysis
Overview : Hypothesis testing can be used to evaluate whether different market segments have distinct characteristics or responses to marketing efforts.
- Null Hypothesis (H₀) : “There is no difference in purchase behavior between different customer segments.”
- Alternative Hypothesis (H₁) : “There is a significant difference in purchase behavior between different customer segments.”
- Analyze purchase data from different segments to identify significant differences and tailor marketing strategies accordingly.
- Targeted Marketing : Develop targeted marketing strategies based on differences in behavior between market segments.
4. Common Hypothesis Tests in Marketing Research
Overview : The t-test is used to compare the means of two groups to determine if they are significantly different from each other.
- Independent Samples t-Test : Compares means between two independent groups (e.g., control vs. treatment groups).
- Paired Samples t-Test : Compares means from the same group at different times (e.g., pre- and post-campaign).
b. Chi-Square Test
Overview : The chi-square test assesses the association between categorical variables.
- Evaluate whether there is a significant relationship between categorical variables, such as product preference by demographic group.
c. ANOVA (Analysis of Variance)
Overview : ANOVA is used to compare means across three or more groups to determine if there are significant differences among them.
- Assess differences in consumer satisfaction or purchasing behavior across multiple product categories or market segments.
5. Challenges and Considerations
A. sample size.
Overview : The accuracy of hypothesis testing results depends on the sample size. Small sample sizes may lead to unreliable results.
Considerations :
- Power Analysis : Conduct power analysis to determine the appropriate sample size needed to detect meaningful differences.
b. Assumptions
Overview : Hypothesis tests rely on certain assumptions, such as normality and equal variances. Violations of these assumptions can affect test results.
- Test Assumptions : Check and address assumptions before conducting hypothesis tests.
c. Interpreting Results
Overview : Proper interpretation of results is crucial for making informed decisions. Avoid overinterpreting results based on statistical significance alone.
- Practical Significance : Consider the practical significance and relevance of findings in addition to statistical significance.
6. Conclusion: Utilizing Hypothesis Testing in Marketing Research
Hypothesis testing is a valuable tool in marketing research for making data-driven decisions and evaluating the effectiveness of marketing strategies. By formulating hypotheses, analyzing data, and interpreting results, businesses can gain insights into consumer behavior, assess marketing initiatives, and optimize strategies.
Despite challenges such as sample size and assumptions, hypothesis testing provides a structured approach to understanding and addressing marketing questions. By leveraging hypothesis testing, businesses can enhance their marketing efforts, improve decision-making, and achieve greater success in a competitive marketplace.
Leave a Reply Cancel reply
Your email address will not be published. Required fields are marked *
Save my name, email, and website in this browser for the next time I comment.
Tips to Create and Test a Value Hypothesis: A Step-by-Step Guide
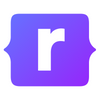
Developing a robust value hypothesis is crucial as you bring a new product to market, guiding your startup toward answering a genuine market need. Constructing a verifiable value hypothesis anchors your product's development process in customer feedback and data-driven insight rather than assumptions.
This framework enables you to clarify the potential value your product offers and provides a foundation for testing and refining your approach, significantly reducing the risk of misalignment with your target market. To set the stage for success, employ logical structures and objective measures, such as creating a minimum viable product, to effectively validate your product's value proposition.
What Is a Verifiable Value Hypothesis?
A verifiable value hypothesis articulates your belief about how your product will deliver value to customers. It is a testable prediction aimed at demonstrating the expected outcomes for your target market.
To ensure that your value hypothesis is verifiable, it should adhere to the following conditions:
- Specific : Clearly defines the value proposition and the customer segment.
- Measurable : Includes metrics by which you can assess success or failure.
- Achievable : Realistic based on your resources and market conditions.
- Relevant : Directly addresses a significant customer need or desire.
- Time-Bound : Has a defined period for testing and validation.
When you create a value hypothesis, you're essentially forming the backbone of your business model. It goes beyond a mere assumption and relies on customer feedback data to inform its development. You also safeguard it with objective measures, such as a minimum viable product, to test the hypothesis in real life.
By articulating and examining a verifiable value hypothesis, you understand your product's potential impact and reduce the risk associated with new product development. It's about making informed decisions that increase your confidence in the product's potential success before committing significant resources.
Value Hypotheses vs. Growth Hypotheses
Value hypotheses and growth hypotheses are two distinct concepts often used in business, especially in the context of startups and product development.
Value Hypotheses : A value hypothesis is centered around the product itself. It focuses on whether the product truly delivers customer value. Key questions include whether the product meets a real need, how it compares to alternatives, and if customers are willing to pay for it. Valuing a value hypothesis is crucial before a business scales its operations.
Growth Hypotheses : A growth hypothesis, on the other hand, deals with the scalability and marketing aspects of the business. It involves strategies and channels used to acquire new customers. The focus is on how to grow the customer base, the cost-effectiveness of growth strategies, and the sustainability of growth. Validating a growth hypothesis is typically the next step after confirming that the product has value to the customers.
In practice, both hypotheses are crucial for the success of a business. A value hypothesis ensures the product is desirable and needed, while a growth hypothesis ensures that the product can reach a larger market effectively.
Tips to Create and Test a Verifiable Value Hypothesis
Creating a value hypothesis is crucial for understanding what drives customer interest in your product. It's an educated guess that requires rigor to define and clarity to test. When developing a value hypothesis, you're attempting to validate assumptions about your product's value to customers. Here are concise tips to help you with this process:
1. Understanding Your Market and Customers
Before formulating a hypothesis, you need a deep understanding of your market and potential customers. You're looking to uncover their pain points and needs which your product aims to address.
Begin with thorough market research and collect customer feedback to ensure your idea is built upon a solid foundation of real-world insights. This understanding is pivotal as it sets the tone for a relevant and testable hypothesis.
- Define Your Value Proposition Clearly: Articulate your product's value to the user. What problem does it solve? How does it improve the user's life or work?
- Identify Your Target Audience. Determine who your ideal customers are. Understand their needs, pain points, and how they currently address the problem your product intends to solve.
2. Defining Clear Assumptions
The next step is to outline clear assumptions based on your idea that you believe will bring value to your customers. Each assumption should be an assertion that directly relates to how your customers will find your product valuable.
For example, if your product is a task management app, you might assume that the ability to share task lists with team members is a pain point for your potential customers. Remember, assumptions are not facts—they are educated guesses that need verification.
3. Identify Key Metrics for Your Hypothesis Test
Once you've defined your assumptions, delineate the framework for testing your value hypothesis. This involves designing experiments that validate or invalidate your assumptions with measurable outcomes. Ensure that your hypothesis can be tested with measurable outcomes. This could be in the form of user engagement metrics, conversion rates, or customer satisfaction scores.
Determine what success looks like and define objective metrics that will prove your product's value. This could be user engagement, conversion rates, or revenue. Choosing the right metrics is essential for an accurate test. For instance, in your test, you might measure the increase in customer retention or the decrease in time spent on task organization with your app. Construct your test so that the results are unequivocal and actionable.
4. Construct a Testable Proposition
Formulate your hypothesis in a way that can be tested empirically. Use qualitative research methods such as interviews, surveys, and observation to gather data about your potential users. Formulate your value hypothesis based on insights from this research. Plan experiments that can validate or invalidate your value hypothesis. This might involve A/B testing, user testing sessions, or pilot programs.
A good example is to posit that "Introducing feature X will increase user onboarding by Y%." Avoid complexity by testing one variable simultaneously. This helps you identify which changes are actually making a difference.
5. Applying Evidence to Innovation
When your data indicates a promising avenue for product development , it's imperative that you validate your growth hypothesis through experimentation. Align your value proposition with the evidence at hand.
Develop a simplified version of your product that allows you to test the core value proposition with real users without investing in full-scale production. Start by crafting a minimum viable product ( MVP ) to begin testing in the market. This approach helps mitigate risk by not investing heavily in unproven ideas. Use analytics tools to collect data on how users interact with your MVP. Look for patterns that either support or contradict your value hypothesis.
If the data suggests that your value hypothesis is wrong, be prepared to revise your hypothesis or pivot your product strategy accordingly.
6. Gather Customer Feedback
Integrating customer feedback into your product development process can create a more tailored value proposition. This step is crucial in refining your product to meet user needs and validate your hypotheses.
Use customer feedback tools to collect data on how users interact with your MVP. Look for patterns that either support or contradict your value hypothesis. Here are some ways to collect feedback effectively :
- Feedback portals
- User testing sessions
- In-app feedback
- Website widgets
- Direct interviews
- Focus groups
- Feedback forums
Create a centralized place for product feedback to keep track of different types of customer feedback and improve SaaS products while listening to their customers. Rapidr helps companies be more customer-centric by consolidating feedback across different apps, prioritizing requests, having a discourse with customers, and closing the feedback loop.
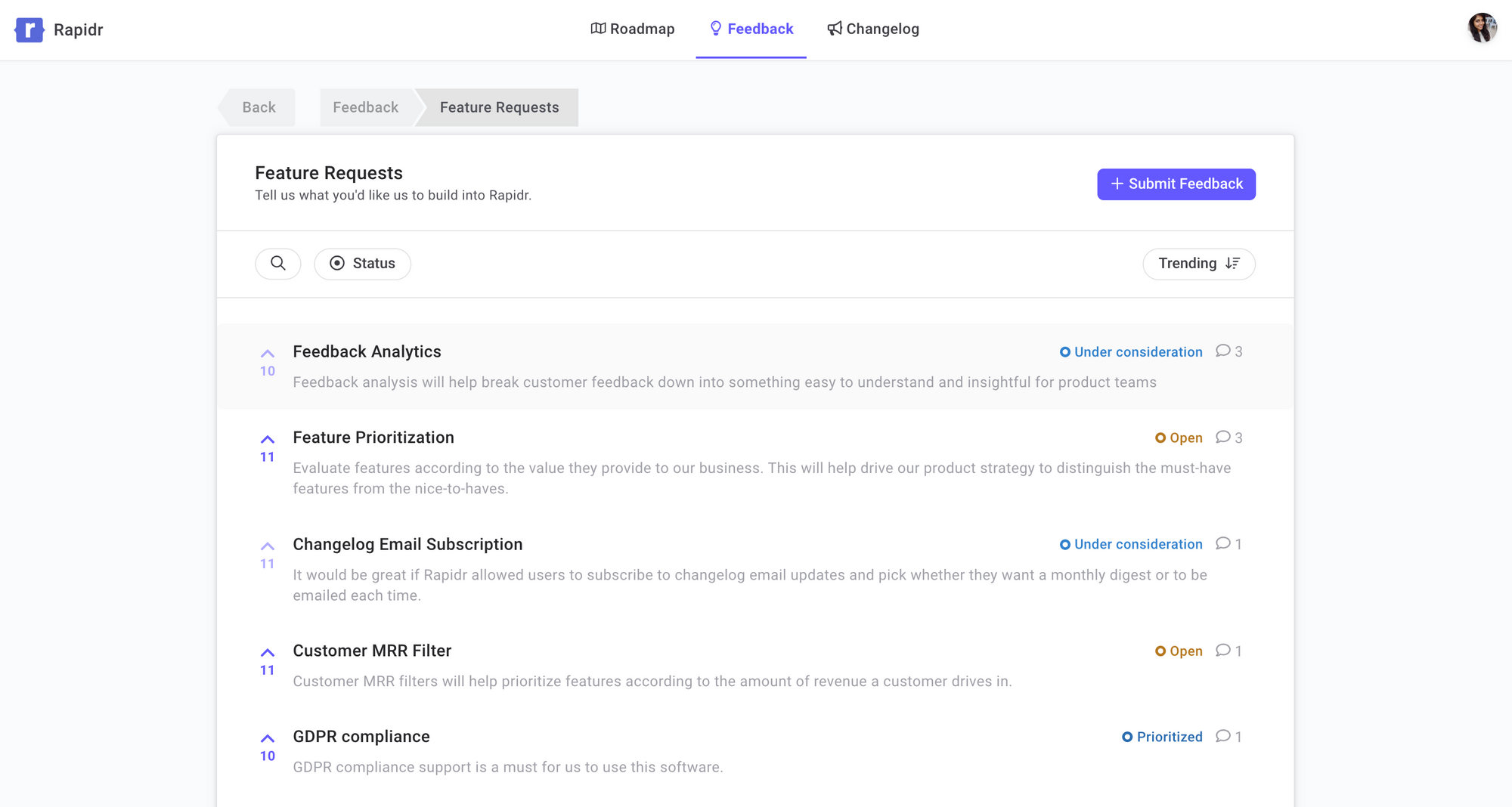
7. Analyze and Iterate Quickly
Review the data and analyze customer feedback to see if it supports your hypothesis. If your hypothesis is not supported, iterate on your assumptions, and test again. Keep a detailed record of your hypotheses, experiments, and findings. This documentation will help you understand the evolution of your product and guide future decision-making.
Use the feedback and data from your tests to make quick iterations of your product and drive product development . This allows you to refine your value proposition and improve the fit with your target audience. Engage with your users throughout the process. Real-world feedback is invaluable and can provide insights that data alone cannot.
- Identify Patterns : What commonalities are present in the feedback?
- Implement Changes : Prioritize and make adjustments based on customer insights.
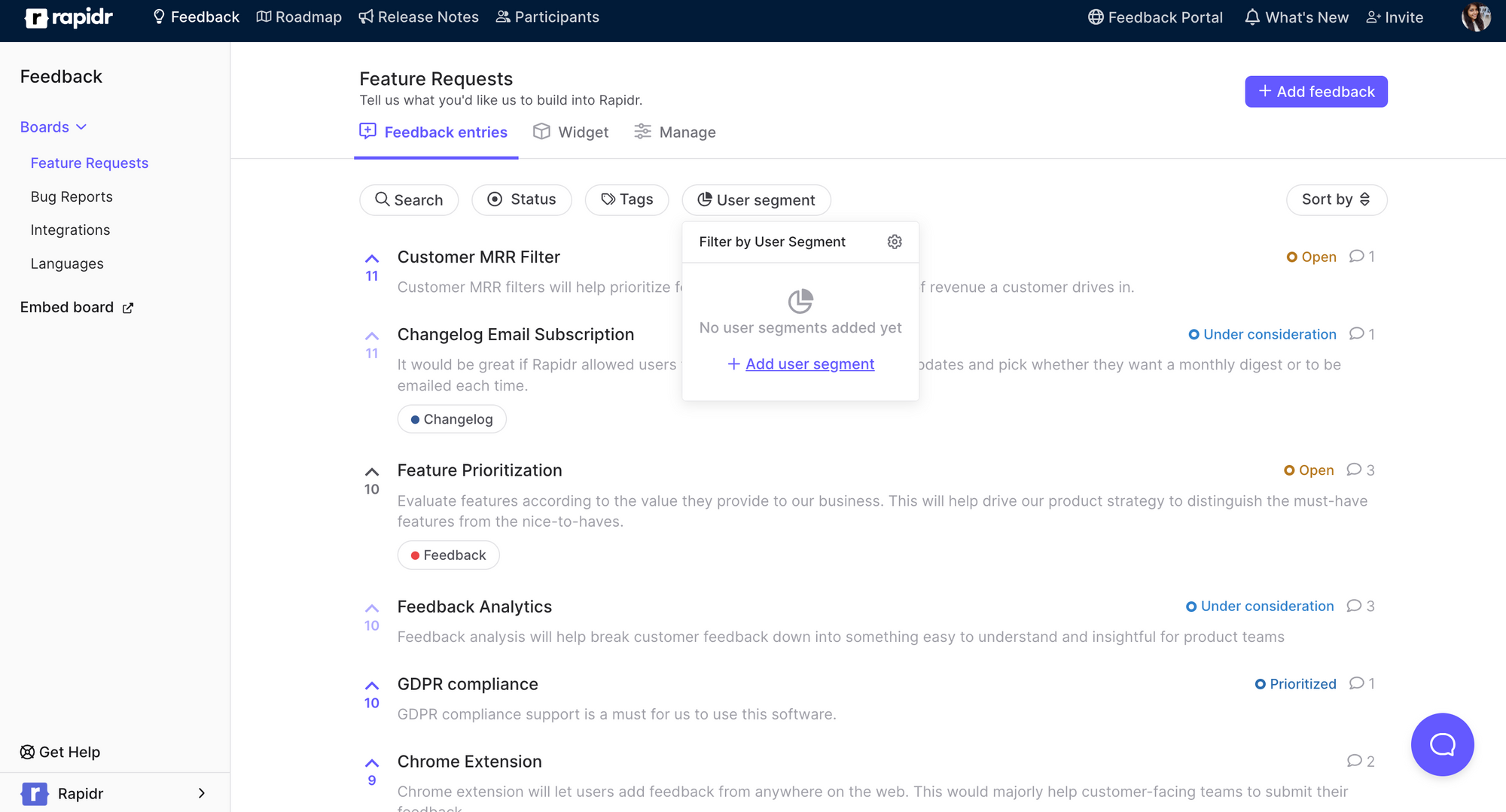
9. Align with Business Goals and Stay Customer-Focused
Ensure that your value hypothesis aligns with the broader goals of your business. The value provided should ultimately contribute to the success of the company. Remember that the ultimate goal of your value hypothesis is to deliver something that customers find valuable. Maintain a strong focus on customer needs and satisfaction throughout the process.
10. Communicate with Stakeholders and Update them
Keep all stakeholders informed about your findings and the implications for the product. Clear communication helps ensure everyone is aligned and understands the rationale behind product decisions. Communicate and close the feedback loop with the help of a product changelog through which you can announce new changes and engage with customers.
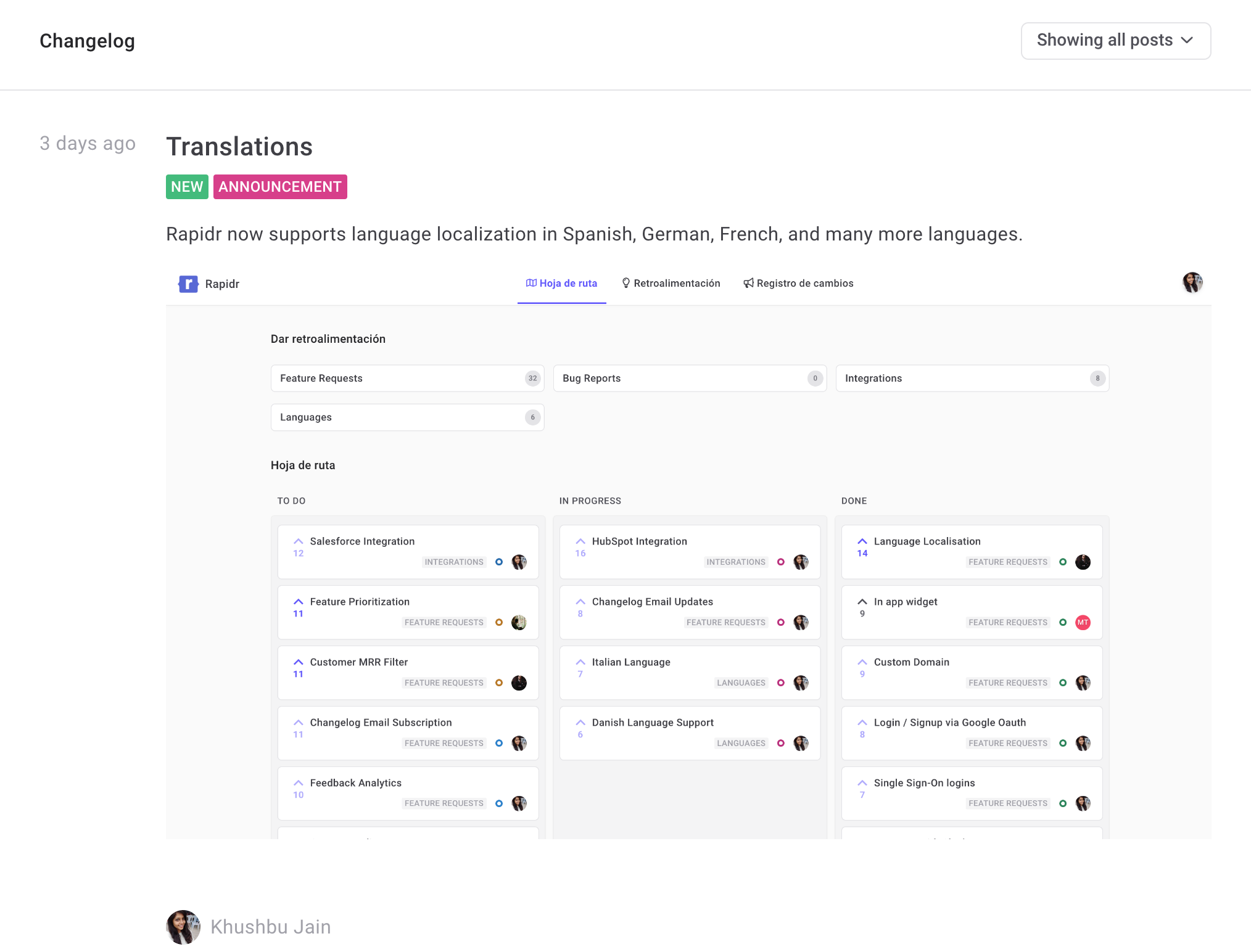
Understanding and validating a value hypothesis is essential for any business, particularly startups. It involves deeply exploring whether a product or service meets customer needs and offers real value. This process ensures that resources are invested in desirable and useful products, and it's a critical step before considering scalability and growth.
By focusing on the value hypothesis, businesses can better align their offerings with market demand, leading to more sustainable success. Placing customer feedback at the center of the process of testing a value hypothesis helps you develop a product that meets your customers' needs and stands out in the market.
Rapidr helps companies be more customer-centric by consolidating feedback across different apps, prioritizing requests, having a discourse with customers, and closing the feedback loop.
Build better products with user feedback
Rapidr helps SaaS companies understand what customers need through feedback, prioritize what to build next, inform the roadmap, and notify customers on product releases
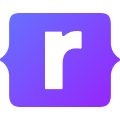

IMAGES
COMMENTS
Hypothesis Group is a consumer insights and strategy agency. We use full-service market research, strategy, and design to help brands do amazing things. Let's work together.
Apr 11, 2021 · With this post, we’re getting advanced with marketing hypotheses, showing you how to write and structure your hypotheses to gain both business results and marketing insights! By the time you finish reading, you’ll be able to: Distinguish a solid hypothesis from a time-waster, and; Structure your solid hypothesis to get results and insights
Jun 5, 2024 · In market research, hypothesis testing plays a crucial role in determining the validity of assumptions and drawing meaningful conclusions. By using statistical methods, researchers can evaluate hypotheses and make informed decisions based on the results.
Mar 17, 2021 · The essential tips on what to do when crafting a hypothesis for marketing experiments; How a marketing experiment hypothesis works ; How experts develop a solid hypothesis; The Basics: Marketing Experimentation Hypothesis. A hypothesis is a research-based statement that aims to explain an observed trend and create a solution that will improve ...
Apr 1, 2024 · Hypothesis testing is a valuable tool in marketing research for making data-driven decisions and evaluating the effectiveness of marketing strategies. By formulating hypotheses, analyzing data, and interpreting results, businesses can gain insights into consumer behavior, assess marketing initiatives, and optimize strategies.
Creating a hypothesis is an essential step in designing and executing effective marketing experiments that can help you optimize your campaigns and achieve your objectives. In this article, you...
Sep 18, 2024 · Developing a robust value hypothesis is crucial as you bring a new product to market, guiding your startup toward answering a genuine market need. Constructing a verifiable value hypothesis anchors your product's development process in customer feedback and data-driven insight rather than assumptions.